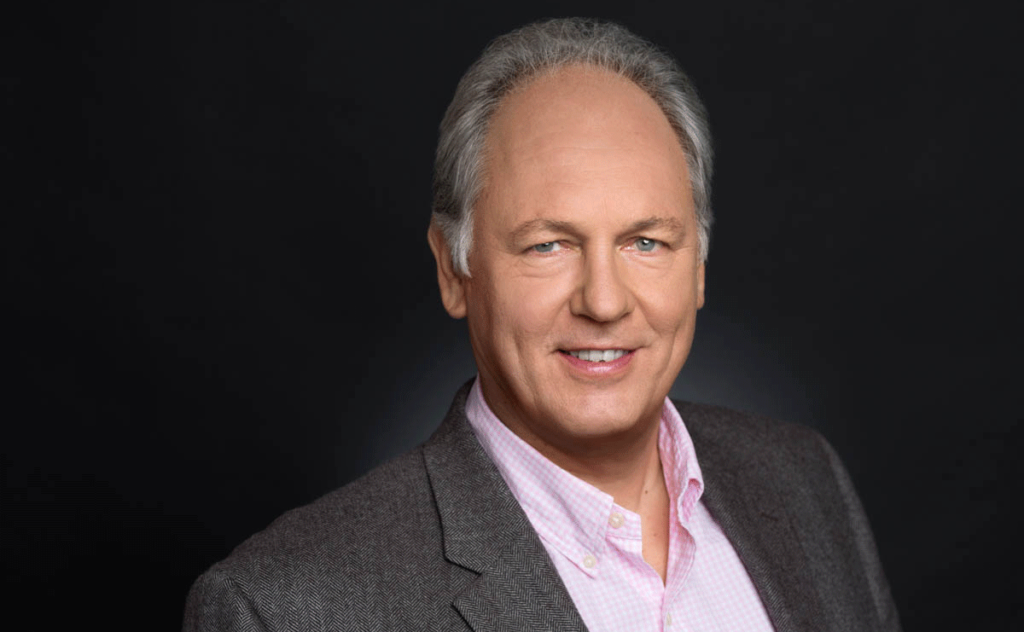
As companies realize the promise of Artificial Intelligence often includes the challenge of integrating highly diverse, increasing complex data sources, the value of semantic graph databases has strengthened tremendously in recent years. Semantic graphs not only enable organizations to seamlessly align unstructured data with semi-structured and structured data—effectively making all data manageable as though structured, but also facilitate interoperability across databases, IT systems, and locations via universal standards.
But these benefits become even more extensible when equipped with the core capabilities of document stores. By augmenting the underlying graph technology with native support for JSON and JSON-LD, organizations can suddenly access a range of possibilities for AI data modeling, querying and developing applications previously impossible.
The heightened utility of a hybrid graph/document store allows organizations to link and access numerous documents, comma separated value (CSV) files, and structured data with a single query, as well as leverage the modeling and formatting flexibility of document stores within the context of their overall data assets. The result is a richer dataset for artificial intelligence, analytics, application development and web services, making data themselves inherently more valuable.
Querying of Structured and Unstructured Data
The analytics capabilities of Semantic Graph Databases supplemented with support for JSON and JSON-LD are remarkable. Documents are typically comprised of unstructured data in the form of natural language text. Using Semantic Graph Databases that provide text analytics capabilities, it is now possible to combine queries over both structured and unstructured data at the same time. Graph-Document databases utilize the semantic linking power of graphs within and between documents. Thus, documents are readily defined according to the semantics that are understood by business users, and also linked to one another through the same semantic concepts that populate the graphs themselves.
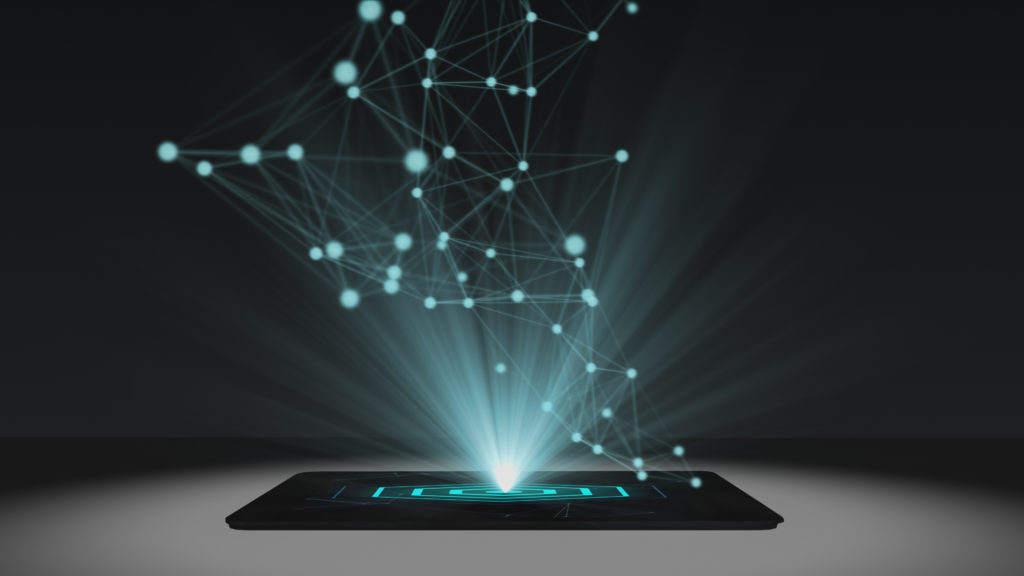
An organization’s internal knowledge graph information can be readily connected to the various data objects in documents, for example. Thus, the scope of analytics organizations can perform in one query is considerably broadened. Queries can not only include unstructured data contained in documents, but also CSV files (useful for machine learning’s feature engineering), semantic metadata, and additional data elements within knowledge graphs related to fundamental business processes.
This approach facilitates the seamless querying of unstructured text alongside conventional data sources, which is critical given the increasing valuation of text analytics to the enterprise today. All of this data is effectively linked via an enterprise knowledge graph for much more contextualized understanding of one’s data and the relationships between them—at which graph technologies excel. Therefore, analytics results are comprehensive and truly indicative of the data organizations have at their disposal for particular tasks.
Model Agnostic
The data modeling capabilities of semantic graph databases enhanced with document database characteristics are almost unlimited. Outside of its use with conventional documents, JSON is universally acclaimed for its ability to support any type of data model or format. It effectively facilitates the notion of schema on read, which is not only useful for on-the-fly data modeling with real-time data sources (involving the Internet of Things, for example), but also for developers and enterprise-wide innovation. JSON allows developers to work with whatever data format or data model that’s most useful for building applications.
Since cutting-edge semantic graph databases now support JSON, developers can use this data in the programming language of their choice while also working with additional data contained in the knowledge graph. Regardless of the complexity of the overarching schema developers are working with, they can contextualize this data and its relationship to other germane data within the graph database. Furthermore, by using JSON-LD they can link this data to those in the knowledge graph for increased application utility or insight into operations during deployment.
Web Services
The other pivotal distinction about providing knowledge graphs with native support for document store formats like JSON and JSON-LD is the utility they provide for web services. JSON is almost the de facto format used for web-based applications, the web itself, and numerous cloud applications. By linking information on the web or applications with JSON-LD, organizations can connect all of this data together for more intricate, effectual applications and web usage.
For example, organizations can join these applications with their knowledge graphs for a network of machine readable data sources, all aligned with the same models buttressing the knowledge graph. This approach is useful for real-time insight into applications, complex data integrations, and application sophistication spanning an array of data points and locations.
Graph Document Stores
The newfound opportunities for fortifying graph databases with document stores are valuable in a host of different ways. This combination increases the flexibility of data modeling, which is imperative for swiftly building applications or incorporating data from real-time data sources like the Industrial Internet. It substantially widens an organization’s network for web applications and services, while producing a similar effect on the amount and diversity of data traversed during a single query. Moreover, it improves document stores with knowledge graph capabilities while also bettering semantic graphs with fundamental attributes of document stores. This combination supports the best of both worlds, leaving the enterprise the clear victor.
About the Author
Dr. Jans Aasman is Ph.D. psychologist, an expert in Cognitive Science and CEO of Franz.com, an early innovator in Artificial Intelligence and provider of Semantic Graph Databases and Analytics. As both a scientist and CEO, Dr. Aasman continues to break ground in the areas of Artificial Intelligence and Semantic Databases as he works hand-in-hand with organizations such as Montefiore Medical Center, Blue Cross/Blue Shield, Siemens, Merck, Pfizer, Wells Fargo, BAE Systems as well as US and Foreign governments.