Enterprises today are in a unique position where it is imminent to digitally transform, stay relevant in the business and realise the business value. While enterprises transform their business with a new set of technological innovations that are driving the digital industry. The adoption of these require the enterprise to be well prepared and ready to successfully, realise the benefits of the digital transformation.
Businesses which have been relying on the legacy technology to run their business and also their day to day operations are adopting innovations in the space of Artificial Intelligence, IoT, Machine Learning, Big Data, Blockchain, Smart cities and more. The existing capabilities, which are legacy in the enterprise today, are on platforms built using the old technologies and not relevant today.
One of the key innovative and trending space is data science, which makes use of scientific methods, Algorithms to bring the value of data, which is lying hidden in an enterprise. Data is the gold mine, which enterprises have to realise needs proper mining to extract the gold nuggets from the same. Data science methods help in implementing and extracting this gold knowledge in the enterprise, which can be found in the formats of structured, unstructured, vast and legacy data.
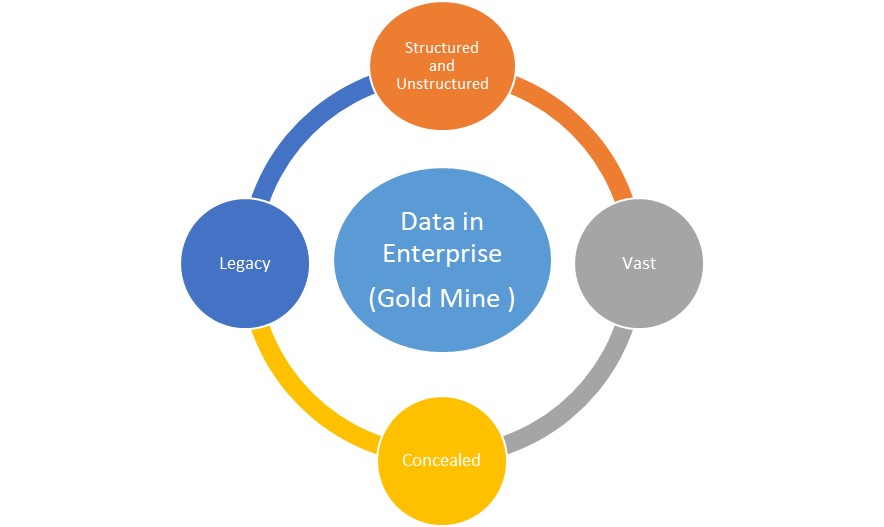
Data science gives a methodical approach to realise the concealed knowledge of data in an enterprise there is a need for a data science platform to achieve the goals of finding right value from the knowledge in the data.
The first steps towards building a data science platform are the foundation, which will ensure that the value of the data is realised in the right way. If not this will lead to the set of gigantic data which cannot be purposed for creating business value from the same. A simple analogy, which I want to drive, is, after identifying a gold mine, to mine the treasured resource there should capabilities in terms of tools, skills, people, and commitment from the business. The same applies to build a data science platform. The commitment must be from the senior leadership with collaboration across the business and technology teams.
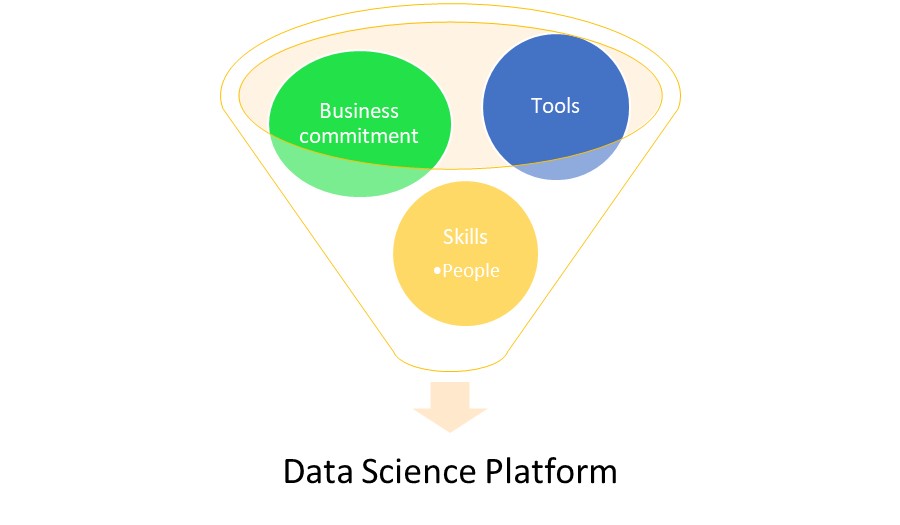
The platform needs to have a specific set of capabilities, which will help the data science team to work efficiently and improve the platform. The data science team will be composed of Data Scientist, Data Engineers, Data Analyst; Business experts will need tools and features that will help in their day-to-day tasks.
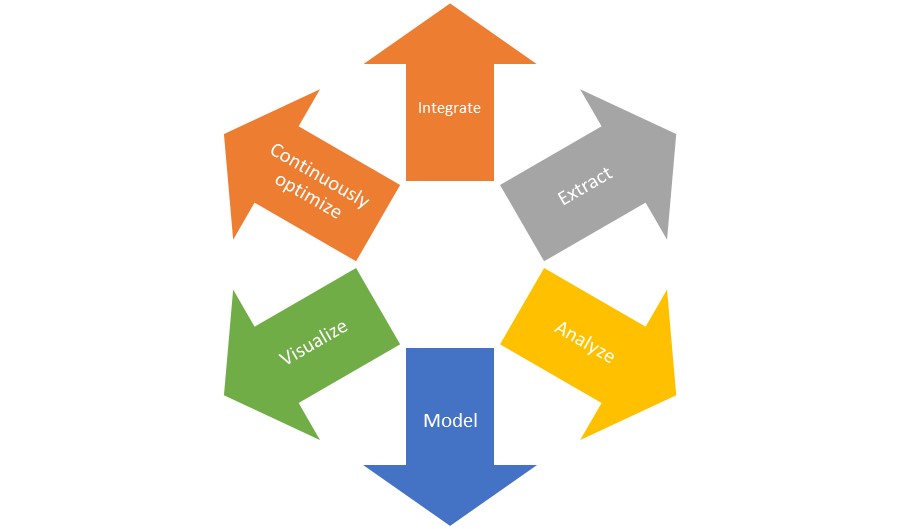
Integrate: Enterprise will have data sources, which are varied, and diverse. The data science platform needs to have the capability to integrate the data inside and outside of the enterprise. While doing so, it should also keep the aspects of security and compliance in strict vigil.
Extract: Data extraction needs to have the right set of tools, which will help in extracting the right set of structure, unstructured and diverse data
Analyse: Features, which will help the data team in visualizing and analysing data for the business value, which is the key golden nugget in the entire data science process
Model: There should support to build models, which will help in building and deploying data across varied resources
Visualize: The platform should help in visualising the modeled data that will help in deriving the business value post applying the data science process. The visualization can be in terms of perspective, Predictive and Cognitive analytics
Continuous optimization: The process of realizing the business value is a continuous process, as more and more data is collected, analysed and modeled, there will be a new set of business value, which will be emerging, and the platform should give the flexibility to do the same
While building the data science platform there are already capabilities, which are available from most of the cloud service providers and more which can leveraged to build the platform in a much quick way. The platform should be able to support
- Data Analysis
- Data science design
- Data Modelling
- Data visualization
- Data Measurement
- Data Exploration
- Data Cleaning
- Supervised and Unsupervised machine learning
- Machine learning algorithms
- Support for languages like R and Python
- Building predictive models
- Operationalizing Models
Building a data science platform is a journey and can start with a basic subset of features and make it operational quickly for the teams to start using the same. The enhancement of the platform can be a parallel process along with strong governance with key stakeholders across the organization to ensure the right feature set are built as part of the platform.