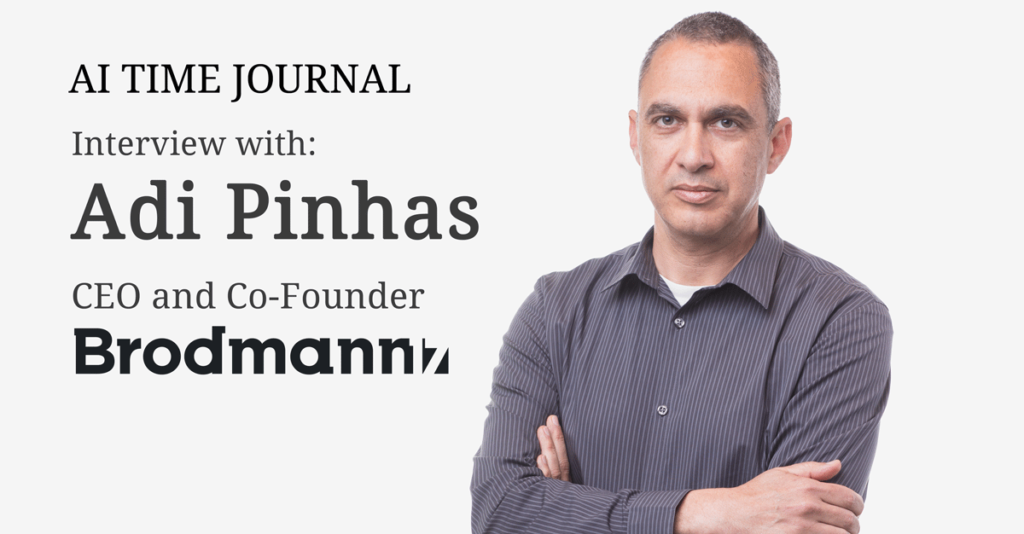
Adi Pinhas is the CEO and Co-Founder of Brodmann17, a software-only deep learning perception technology provider bringing Advanced Driver Assistance Systems (ADAS) and automated driving to the mass market.
The company, headquartered in Tel Aviv, has recently raised $11 million in a Series A funding round and is now expanding its operations in Detroit and Germany.
Prior to co-founding Brodmann17, Adi Pinhas led a research team at Intel and started two successful companies: Vigilant Technology in 1998 and JustVisual in 2006.
What is your background and how was Brodmann17 started?
My background was always in computer vision and Brodmann17 is the third company that I’ve started. My previous two companies were also computer vision-based for different applications and different markets. In 2012, while doing computer vision, we encountered this new technology of deep-learning and, like everyone else, we saw that it’s the best algorithm for computer vision. From that point forward, we started to use only that for these types of applications.
My co-founders and I worked at different companies at the time, developing deep-learning for them and of course it was all implemented in the cloud. We saw the remarkable results that you can achieve in the cloud and we knew that the next frontier would be to take AI and deep-learning to Edge devices, where there are big challenges. There are not as many resources as there are on the server side. Initial implementations of deep-learning couldn’t care less about resources. It was so exciting to get these amazing results that initially no one cared too much about the associated compute cost.
So we started Brodmann17 around the idea that we can develop deep-learning that is as good as we saw before, but able to run on Edge devices.
What challenges did you face developing this technology, and how did you overcome them?
The big discovery of deep-learning, like many people are now saying, was nothing new. We knew this concept already during the ’60s. What was discovered was that if you apply and create really large, very deep neural networks, then you start to get amazing results, which are not just better, but a lot better.
The initial approach was “let’s put in a lot of resources and then we’re getting remarkable results.” Some academic benchmarks that always had over 30% error rates suddenly became less than 1%. The big challenge with running these algorithms on Edge devices was how to use fewer calculations in order to achieve the same results, and it was very hard.
Today we know it’s practically impossible to modify these algorithms. Because these algorithms were created in this way, we had to reinvent deep-learning. We created a completely new deep-learning algorithm and this algorithm was designed from day one to reuse calculations. We designed our neural networks in a way that allows certain parts of the neural networks to reuse calculations that were previously made.
We had to reinvent deep-learning. We designed our neural networks in a way that allows certain parts of the neural networks to reuse existing calculations.
Adi Pinhas
Our neural network is large and accurate, but the amount of unique calculations are only 5% of the total calculations usually required. This is how we reduce calculations, thereby reducing power consumption drastically. Instead of using one of the open-source neural networks like everyone else and trying to optimize it, which allows you to achieve probably around 3x to 4x improvement at most without losing accuracy, we created neural networks from scratch. When we did this, it was possible to design them in a very different way to reuse calculations. And that was the innovation; that was the starting point that we worked on for almost two years.
Tell us about the Brodmann17’s ADAS products
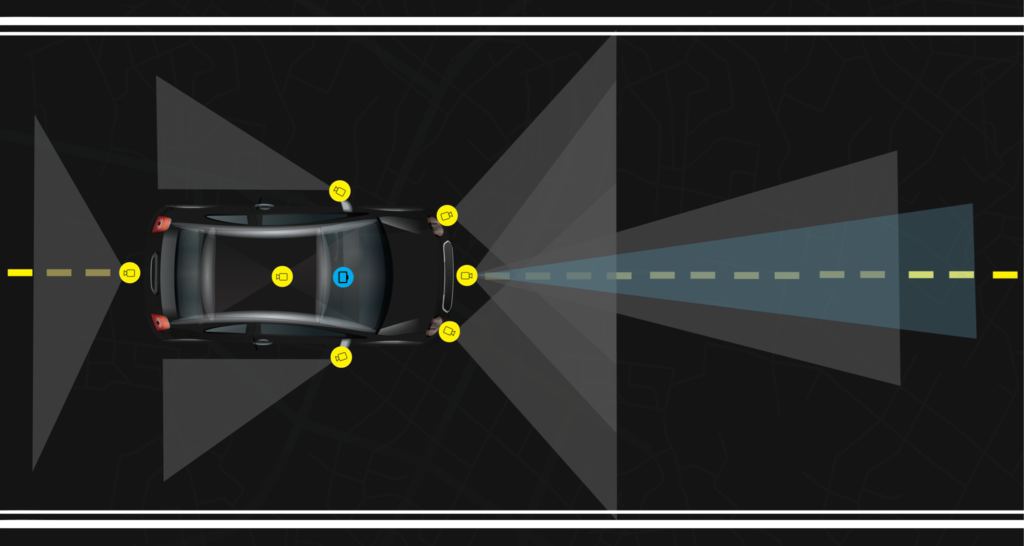
The media is always talking about autonomous vehicles and different levels of autonomy. But the real revolution today is not in autonomous driving, it’s in the Advanced Driver Assistance Systems (ADAS). ADAS features include pedestrian collision warning, forward collision warning, automatic emergency braking, automatic parking, and so on.
These features are sometimes just a warning signal to the drivers, or sometimes take action like hitting the brakes. These are the features that are creating the real revolution today. They are being sold by the tens of millions and they have proven to save lives and reduce accidents. This is why we see the market looking today for ADAS solutions and this is our main focus.
We do see automated driving and autonomous driving down the road. We expect that more will happen around that next year. But even the most optimistic estimates are talking about tens of thousands of autonomous vehicles in a few years, whereas with ADAS we are talking about tens of millions.
In addition to automotive, is the technology that you developed applicable to other domains?
Our algorithm is very general. It can show improvements across different domains and across different processors, like the low power processors that you usually find in Edge devices. But the algorithm also shows that you can get this 20x advantage on servers.
That was one of the challenges of the company. When you start a company with such a widely applicable technology, you need to make a decision, because it’s way too much for a startup to take on. We saw that on the server side, our technology can deliver an amazing cost reduction.. For cloud, forget about 20x, even 2x is a great discount. If you can reduce your cloud expenses by 50%, it’s great.
But for vehicles and Edge devices we are not just delivering a cost reduction. We are delivering a complete game-changer. So we decided to focus on that.
For vehicles and Edge devices we are not just delivering a cost reduction. We are delivering a complete game-changer.
In the automotive space, people frequently think about a car as something very powerful in terms of power and cost and so on, but that’s not the case. The processors that are implemented in the vehicles are significantly weaker than the big processors that you see being developed every day from the likes of Intel and Nvidia on AI; these chips do not exist in the automotive space.
How do you position your company in the automotive space? What’s your advantage over the competition?
The challenge in the automotive space is how to make it work when you have pressure around the cost of the processor and the size of the computer. If you search online, for example, for a Tesla autopilot computer, it’s big and costs a few thousand dollars. This is really not practical for the mass market. The mass market needs solutions that are cheaper than that while achieving the same or even better levels of accuracy.
This industry never compromises on accuracy and precision. But they also have strong pressure around the price. Because when you look at these features that are promoting safety and comfort, like auto-parking, of course people like them, they’re great features. The main market challenge for the mobility industry and the automakers is how to close the gap between what people are willing to pay for these features and how much it costs to implement them. Today they are too costly and this is why you mostly see them in premium vehicles, or sometimes they have reduced capabilities. We see now that automakers are trying to understand how to bring these features to the mass market and this is where we have a big advantage: we can work on the low power processors that they select.
This is based on what we’ve recently seen when automakers need to create practical solutions that they can deploy with low power processors: in the cases where we got the deal and signed the development agreement, we were the only company that was able to do that. The amount of resources or the processor that the automaker chose for these applications was relatively weak and we were the only company that was able to accomodate for that.
I think that this is going to be the main advantage of our company. And of course, with time we’re going to gain more and more experience around these applications.
What is your business model?
Usually in this space, when you license a software, it’s royalty based. So it depends on the number of vehicles that are going to use this technology. You see different business models now, and many of the one-time payment business models are being replaced by a service. The automakers are also interested to see how they can change the traditional business models around these new features that they are introducing to the markets.
How long did it take for you to complete the POC?
The POC was around four months. And what these companies are looking to see is the quality of the algorithms. They have their own internal tests, so they don’t use the academic benchmarks. They have a far more specific test that they’ve developed, so the POC was testing the quality of the algorithm and of the test videos that they have.
The second part was an evaluation to see that it could be implemented under the given resource conditions. And once we show that we can do both, then we sign a development agreement that usually offers an additional four to six months to deliver the final software, so that they can start their testing. And if they need to, they take it to different regulations and so on.
When we meet companies, automakers or Tier-1 suppliers that are selling to the automakers, usually they have already made a decision about the specifications of the system that they’re developing – he processor, camera, applications, features, and so on. Their planning staff do this and what we deliver for them is the code part for this system.
Do you sometimes collaborate with the hardware makers?
Absolutely, we are in close contact with many chipmakers. You can see some publications that we did in the past with Arm from Japan, companies from the UK, SoftBank in Japan, and with CEVA DSP, and so on. We are working very closely with these companies. A few of them are using our code as a reference design and even demoed this at CES in January. For them, it’s a big advantage to be able to work so closely with software companies like us.
Tell us about the Brodmann17 team.
Most members of the team are machine learning engineers. We have about 20 researchers who focus on machine learning. We believe that if you get the algorithm right, then everything later on is a lot easier. The development of the software, the development of the hardware, everything has become easier if you have the right algorithm – that is, if you have an algorithm that is lean enough.
We believe that if you get the algorithm right, then everything later on is a lot easier.
This is where we focus most of our resources. We have 10 people on the product and the business side or the customer-facing side of the company, and now that we recently completed our Series A funding round, we are going to build up this side of the company – the customer-facing and support side. We are hiring people in Detroit, Germany, and more.
Is hiring a challenge?
Finding talent in AI is highly challenging today. This is why we have a recruiter whois solely devoted to that. Luckily, people really like the research we are doing over here. So when we show them some of the results and the work that we are doing, it makes them want to join us very much.
What would you say was the biggest challenge of the company so far and moving forward?
It’s a moving target. I think that creating the team was a challenge and we needed to spend a lot of time to see how to build it right in order to maintain the capabilities of the core team and be able to continue to support this very complex research. I think the challenge for us now is to productize the technology. Everyone is looking at AI a bit suspiciously and thinking about how to make a product from these technologies, especially when you go into the automotive space.
What is the company’s biggest achievement in the last 12 months that you’re particularly proud of?
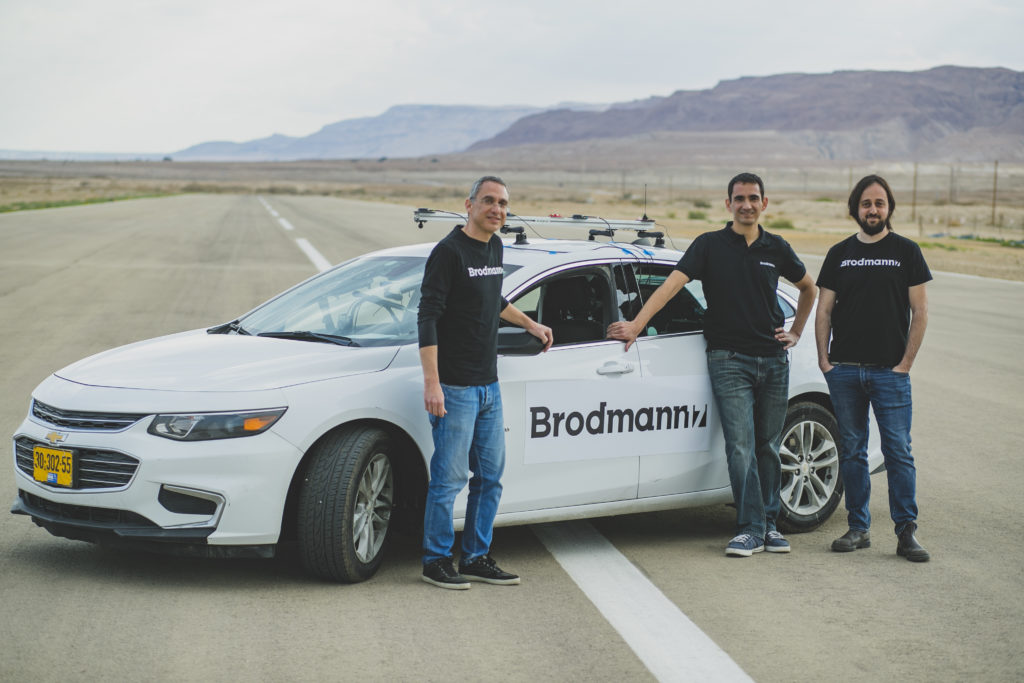
I think that signing development agreements with automotive Tier-1 companies is a huge achievement. For a 2-year-old software company to be able to develop the capability to have a conversation with automakers and show them that our technology is good enough and that we can create products for them that they can integrate in their vehicles is a huge achievement for our team. Everyone here working on the technology and the product has done an amazing job.
Even when you are a 20-year-old company, to connect with an automaker is a big challenge. For a 2-year-old company, this was a remarkable achievement that we celebrated.