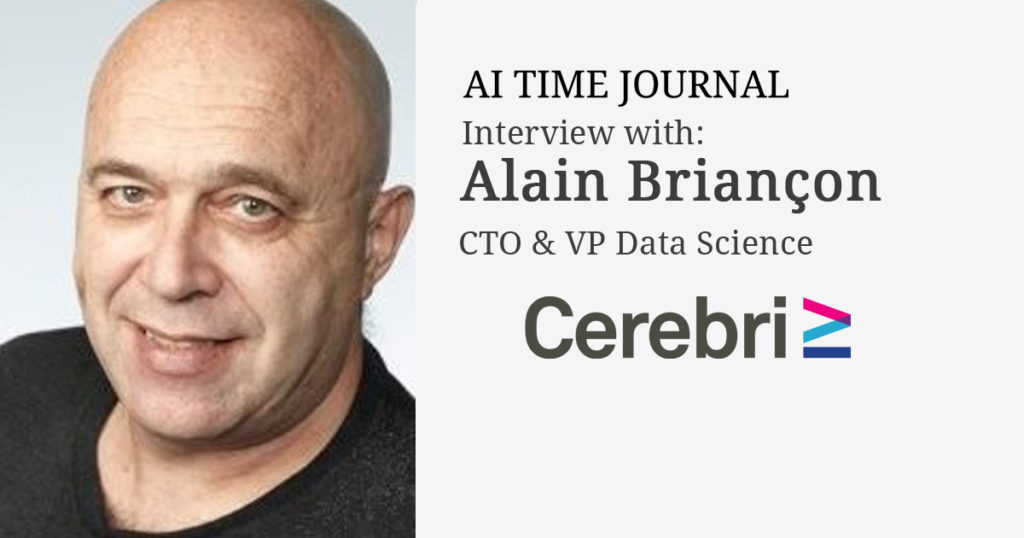
Dr. Alain Briançon is the Chief Technology Officer and VP Data Science of Cerebri AI, an AI platform company that helps enterprises better understand what their customer needs are through data. Their technology is applied to a wide range of industries such as automotive, financial, telecommunications, and travel.
What is your background and what made you join Cerebri AI?
I am an engineer by training. I have an MIT PhD in Electrical Engineering. I am an avid history, opera, and movie buff. I am a serial inventor, with 230 and counting patents worldwide, in fields ranging from wireless tech, to mobile apps, chemistry, internet of things, security, machine learning profiling, and most recently artificial intelligence. After years in the corporate world (mostly in Telcos), I jumped to the start-up world to try things out.
Cerebri AI is my fifth startup, the fourth one where data Science and AI are key components. I am hooked. Why Cerebri AI? Because after 20 years of trying to recruit me, Jean Belanger, our CEO, made the case that we could finally provide the non-icky personalization of customer experience at scale. That was worth a lot. I did the technical due diligence (silly given that M12 – the venture arm of Microsoft, the largest AI company in the world had just invested in Cerebri AI). We decided that we would open an office in DC, where I reside and where there is tremendous talent in IT, AI, and database technologies (ask Amazon what they think).
Joining Cerebri AI was one of my best decisions ever.
What is Cerebri AI’s mission?
We have been too busy creating insight and value for Fortune 500 companies to have an official mission or vision statement. But if I had to put one together, I would start with the fact that we put the customer at the center of the enterprise in a meaningful way, leveraging the massive amounts of customer data recorded by companies.
AI is, at its core, an ingredient technology. Cerebri AI is no different, albeit a better ingredient. Our ingredient is smart personalization. Our Mission “To personalize you”.
Who are Cerebri AI’s customers and how do you create value for them? What challenges do they face, or will they face in the future, that Cerebri AI can help them overcome? Are most of your customers in a particular industry, and if yes, why?
To date, we have focused on the automotive, financial, telco and travel sectors, but the Cerebri Values platform has universal applicability. Our current clients include a leading North American bank, a central bank, a top 10 global auto OEM. We have proof of concepts going with one of the largest wireless operators in the world, one of the largest banks in the Middle East, a major software company and an insurance company. We reach more customers through our great partnerships with Microsoft and MasterCard.
We help our customers understanding their customers in a very unique way, which allows them to better serve and grow.
We help reduce the manual processing and costs around customer measurement and reduce the cost and complexity of creating Next Best Action{set}s.
Walk us through Cerebri AI’s products, and what problems they solve. Which product are you currently focusing the most on? Which product do you think is creating most value for your clients, and why?
Our proprietary Cerebri Values CX Platform measures customer engagement and produces improved financial returns using RL-powered Next Best Action{set}s.
All businesses are challenged with having a true understanding of their customers and knowing what to do with them next. The larger the business, the larger the challenge.
We help reduce the manual processing and costs around customer measurement and reduce the cost and complexity of creating Next Best Action{set}s. Our solution addresses the following pain points:
- Inconsistent customer measurement across channels and business units.
- Reduction in the number of AI models required to produce an action.
- Reduction in the cost of model re-training.
- Better communication timing.
- Better alignment of messaging and offers to the appropriate customers.
Cerebri AI provides behind the corporate firewall (PII is a core issue).
One of the crown jewel of machine learning is reinforcing learning. We have found that changing only the order and timing of marketing communications can improve car sales by more than 25%.
How do you measure the performance of your product?
We measure customer engagement, or CE, systematically across all customers. This takes the form of a score between 0 and 100. We then use this measure to drive both customer engagement and customer success (increased financial results from selling more product and services – such as cross-selling, up-selling, acquiring new customers, etc.).
These results are provided in terms of scores or lifts (change in key metrics), that are predicted on actions selected by users from actions recommended by the platform.
With Cerebri’s v1 platform customers were seeing 5x to 7x increases in conversions to sales on email, SMS, and direct mail campaigns. They were seeing 30% increases in response rates for call center interactions. In financial services, we started with the risk element in customer journeys and increased automated loan origination by $1B/year without a substantial increase in risk.
One of the crown jewel of machine learning is reinforcing learning. We have found that changing only the order and timing of marketing communications can improve car sales by more than 25%.
Tell us about the Cerebri AI team.
Opinionated, smart, and direct. Cerebri’s executive management has been around the block a couple of times and has the battle scars of enterprise software to prove it. We have to move quickly, and few decisions do not affect the entire enterprise. That means very direct reviews of customer situations, algorithms or user interfaces. We are an ambitious team and the successes we’ve had started to vindicate our approach.
How many data scientists or machine learning engineers do you have in your team?
We have about 45 people in the technology team, which spans from data engineering, data science, back-end engineers, and full-stack engineers. We need this mix of talent as we develop models with an eye to real-time software implementation and we span the pandas/python/pySpark/Scala continuum.
One key element of our design is a model orchestrator and utilizing core models that are independent of the dataset provided by customers. This requires very systematic modeling, development, and deployment strategy.
We have one team spread across three cities: Austin, Toronto, and Washington DC. We use the separation as an advantage to force written documentation of projects and results. We can also hire talent more readily that way.
Which AI technologies are you experts on and are you leveraging the most?
We are experts in developing Object-Oriented Modeling (OOM) that can be characterized as the 3rd generation autoML technology. OOM does for AI, what Object-Oriented Programming did for software in the mid ’90s.
We have four core models object dubbed pillars that cover propensity, affinity, next best action sets, and engagement. They can be adapted automatically to many datasets.
In these pillars, we use typical supervised and unsupervised techniques, albeit with very proprietary optimization and feature engineering.
The other technology suites that we use and build on are a conditional contribution and causality tools, such as SHAP, counterfactual analysis.
Generally speaking, we stay away from pure deep learning implementations, apart from for feature engineering.
We are an ambitious team and the successes we’ve had started to vindicate our approach.
What skills do you look for when hiring data scientists?
Smarts and curiosity. It is easier to pick up smarts when hiring directly out of school. That is where we do the majority of our hiring. Our interview process is thorough. It involves typically a technical test, two technical interviews, HR, me and, at times, our CEO.
Anyone involved in the process has veto power. No override. Smarts is understanding the limitations of machine learning. Providing too many significant digits is not sign of smarts (and is likely to get your resume rejected from the get-go). Everyone ‘sky learns’, but you need to be able to explain why you do it.
Curiosity is a key differentiator. Do you read? Do you paint? Are you willing to fail and admit you got it wrong? Not all our hires have long academic careers in machine learning, most don’t. Changing a degree or focus during one’s studies is a sign of strength and curiosity.
Quantum entanglement might not help machine learning, but the duality wave-particle for light does (well it did). When you are curious and smart, you realize there is more than one way to skin a cat, who might be dead and alive at the same time – clearly, we inspire ourselves from quantum mechanics.
What are the biggest challenges that you are currently facing at Cerebri AI?
Convincing customers that we do not need ALL of their data to move the needle.
What are Cerebri AI’s biggest achievements in the last 12 months?
We call it SHARP. SHARP is our reinforcement learning (RL) architecture, which we use to design next best actions for businesses. SHARP moved the state of the art for RL way forward. SHARP enables a business to take suitable actions to maximize reward, which could be a customer buying a car, someone taking credit based on a lender’s terms or renewing a wireless contract). The rewards are KPIs for the enterprise.
SHARP learns from historical and life data, across an entire set of a customer’s interactions with a single business, and accounts for the business’s r timely (or untimely) responses. Unlike how RL is used to play games, such as chess or Go, where time is not a factor. SHARP accounts for delays in action. And, as we know, time matters – a lot.
Talking of times, best actions (Bas) have typically been rules based (if the customer is female and her last purchase was less than $300, do this). They are driven by a single KPI, one action at a time. SHARP determines the best action, the next best action and the one after that; and figures out the impact on all KPIs at once. No longer, can you drive to impact sales in Q3 and ignore the impact on churn in Q4. SHARP is, well, sharp.
Which AI-related technology trend do you think will have the biggest impact in your industry in the coming years?
Federated learning, enabled by homomorphic encryption, that is tokenized on a per use case basis to solve the privacy conundrum.
Real time voice interpretation and translation.