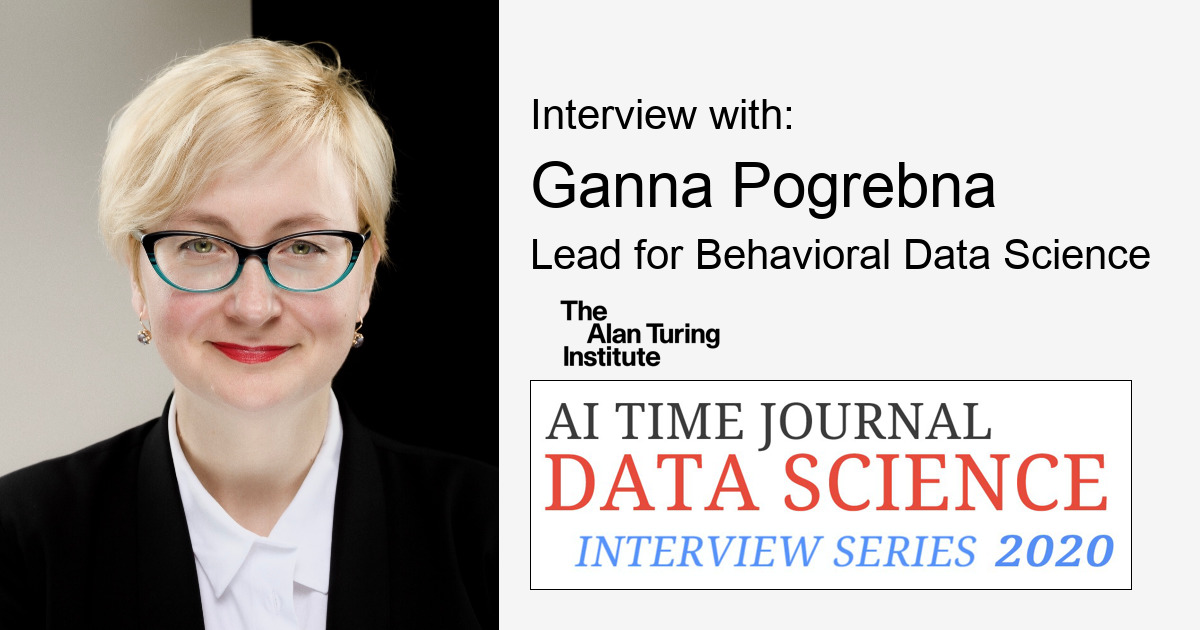
Ganna Pogrebna is a researcher at The Alan Turing Institute. She hosts a behavioural data science podcast and has recently been nominated as one of the AI Time Journal inspiring data scientists to follow in 2020.
We thank Ganna for taking part in the Data Science Interview Series 2020 and sharing several insightful reflections from her experience, including:
- The importance of talking with people from different fields to come up with out-of-the-box solutions.
- Her thoughts on the diversity and inclusivity of the data science field.
- Her activities as a podcast host.
1. At what point did you realize that you wanted to pursue a career in data science, and how did you get into it?
Unlike many people, I got into data science by complete accident. I started my career as a decision theorist, working on quantitative models of human behavior. I worked in many different universities including Columbia University in the City of New York (USA), University of Warwick (UK), Humboldt University (Germany), etc.
My initial work was in behavioural science rather than data science. Much of my work was about writing mathematical models trying to predict human behavior and then testing these models in the laboratory. The way this worked was I would write a model of human decision making, and then would invite study participants to the laboratory, where they would be making a series of decisions. I would then use the data from those laboratory sessions to see whether the model worked or not. If it did not work – I would write a different model.
While it was exciting to work on predicting human behavior, the laboratory research usually involved several hundred participants; and I always wanted to check whether my theories would work at scale and would deliver value in the real world.
So, in 2013 I managed to get a job at the Warwick Manufacturing Group (University of Warwick), where I could work with businesses in the UK on many projects in consumer choice, digital transformation, and AI using large-scale datasets. My first project looked at using smart-home sensor data to predict consumer decisions. That project changed my life and I knew from that moment on that data science, and, specifically, behavioural data science, is what I wanted to do.
2. How is data science used to create value in your current project(s)?
Much of my work is about understanding people’s preferences. Data science creates value in my projects in 3 ways:
(1) As I work on hybrid models between decision theory and data science (such as, for example, Anthropomorphic Learning modelling approach, which I have been developing for the last 18 months), data science allows me to develop new knowledge.
(2) Data science allows me to solve problems at scale – for example, recently my team has made significant progress in making suggestion systems more useful to consumers using Anthropomorphic Learning;
(3) Finally, unlike other tools, data science allows me to create forward-looking models. For example, using traditional marketing we can look at an existing product/service and predict what type of consumer would want to buy that product/service (in a way, we back-engineer preferences to products and services). With behavioural data science, we can write forward-looking models – for example, we can predict what features of a product/service a particular consumer would want to have tomorrow and then not only create this personalized product/service, but also deliver it to consumers using mass-customization tools.
In a nutshell, to me, data science in general, and behavioural science in particular, allow to see how data and data-driven insights propagate through the entire supply chain or business model to produce more value for the business as well as increase customer satisfaction.
3. What is one of the best investments that has propelled your data science career the most?
In my case, the most important thing was to develop my network. Through this network, I met people who helped me understand what skills I need to have in order to solve the problems I want to solve. And in my view, the best thing to do is to talk to people who are not working in my field. The best ideas, results, collaborations, and projects usually come when you think outside the box. You do not need to invest much time, money, or energy into this. The only thing you need is the desire and willingness to listen and understand a different point of view.
In my view, the best thing to do is to talk to people who are not working in my field
4. How do you keep current with the new developments?
I am fortunate to be working at one of the top places in the world for Data Science – the Alan Turing Institute. So, my first place to find out about what is going on is the Alan Turing Institute, its website, workshops, conferences, and other virtual and face-to-face events. The second place is arXiv, where I always read the latest papers in data science, machine learning, and AI. Only by reading the original papers you can judge how valuable proposed model innovations actually are. Finally, our special interest group in behavioural data science at the Alan Turing Institute as well as the Data Driven Chat podcast, where we invite the coolest people in data science and related fields is a source of constant inspiration and learning for me.
5. What are the top challenges you currently face as a professional data scientist, and how do you go about tackling them?
One of the main challenges for me is always about learning new tools, or, finding the time to learn new tools. There is always something new to learn and it is easy to miss, as there is a lot of information out there about data science.
For example, I now realize that my knowledge of Python is not enough for everything I do, so I am learning Julia and it takes time to do that. Another important challenge for me is thinking about how to make sure that all models and tools I develop use people’s data in a responsible way.
It is very easy to hurt people with data science tools, so I always try to anticipate the potential adverse effects of the behavioural data science models I develop.
6. How important is the domain knowledge of the business/industry you’re in as a data scientist, and how did you acquire it?
I am an academic, so I often work as a consultant to businesses, delivering behavioural data science solutions (for example, my most recent project was developing a personalized chatbot technology). Nevertheless, the business/industry/domain knowledge is extremely important. I always work with people, who have this knowledge in organizations I consult. The main advantage of having them on board is that (i) they understand the problem that needs to be solved and can formulate the problem statement for a data scientist; (ii) they know where the data comes from, how valuable/useless these data are, and, most importantly, what different variables mean.
Many models we, as data scientists, work on, aim to solve real-world problems. Therefore, understanding how data science can help in a particular business context is important. Equally, it is important to have a person, who knows the organizational data well – our models work well only if they have great training sets, so having an understanding of data in the business context is key to project success. I do not think that a data scientist needs in-depth domain knowledge, but I do think that the project team should have at least one person who has this knowledge and can help guide the work.
7. What unusual or absurd thing do you practice or advocate for in your profession as a data scientist?
Several things come to mind. First, I have over a dozen of GitHub pages and I never use my real name on any of these pages. The main reason for this is that I have written some code and developed some software in the past, which are now used by many people. And the problem is that once you develop something, people expect you to maintain it. Yet, I always want to work on something new. I think many people do not appreciate that open source recourses such as GitHub are there to make sure that people collaborate on projects. They are not there to bombard the original developer with thousands of emails requesting to “update” the code/software. The point is – if you are the original author of the code or software, which you decide to make open source, you no longer own it – it belongs to the community.
Another thing which I think is unusual, but very important, is to explain what you do in simple ways – for this reason I have created my YouTube channel, where I try to talk about data driven science and projects in simple terms, which any person will be able to understand.
8. What inspires you about working in data science?
I love two things: (i) as a behavioural data scientist I work on many different projects (from how people make purchases in the supermarket to how astronauts make decisions under risk and uncertainty in space) and this is really cool; as well as (ii) diversity and inclusivity of the field, where we have thinkers (who understand the “black box” data science/AI models conceptually); do-ers (who apply “black box” models in practice) and developers (who change “black box” models, perhaps even working on making sure that the models we use are no longer “black boxes”).
9. What advice would you give to someone who wants to get into data science today?
I would say that two most important things you need are imagination and a desire to learn. If you have imagination, you will be able to formulate interesting problems. And once you have formulated your problems, if you are willing to learn, you will get the skills to solve these problems.
What advice should they ignore?
There will be many people in your career, who will tell you that something is impossible and you should not try it. If you listen to this advice – you will stay where you are and will not develop. I like this quote from James Cameron: “If you set your goals ridiculously high and it’s a failure, you will fail above everyone else’s success”. Essentially, do not give up only because someone told you so. Equally, do not give up if you fail the first time around – try again.
10. What have been the most relevant breakthroughs in data science and machine learning in the last 1-2 years, and what trends do you see emerging going forward?
My answer will be very biased towards my personal preferences, but I think in recent years major breakthroughs were (i) XAI models, particularly models which help understand machine learning predictions (i.e., help to “unpack” the black box predictions) – e.g., LIME model and similar techniques; and (ii) the emergence of behavioural data science as a field – it is cool to mix and match theories of human behavior with machine learning models!
11. Tag one or two data scientists that you would like to see answering these questions.