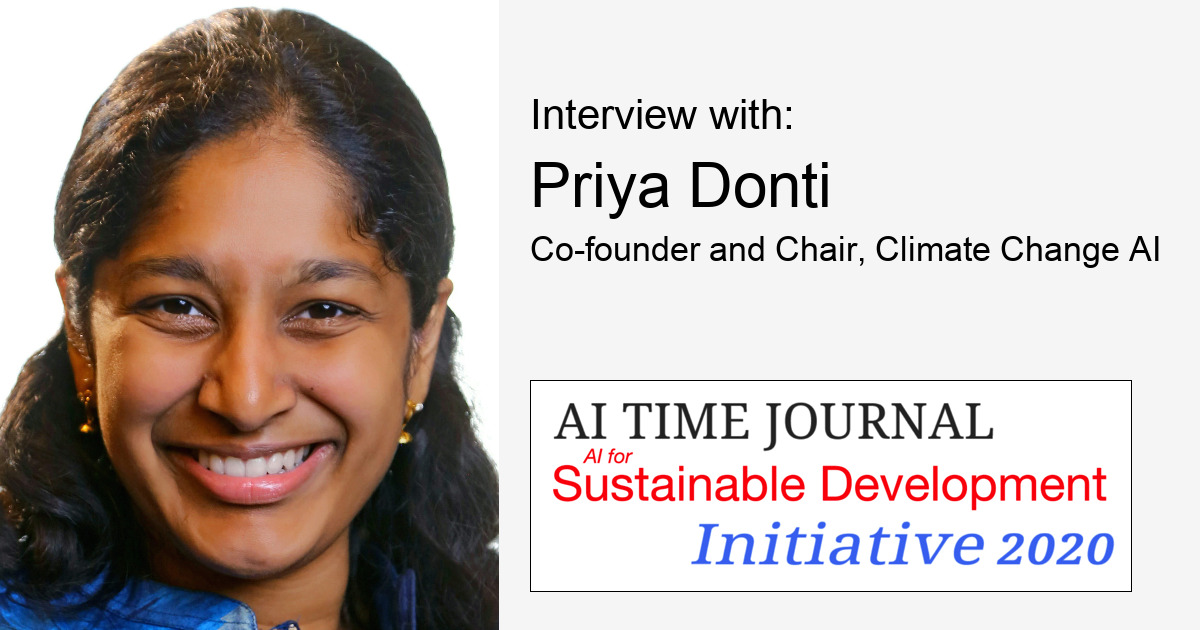
We thank Priya Donti from Climate Change AI for taking part in the AI for Sustainable Development Initiative. It was an immense pleasure understanding her opinion on the current situation of climate change and how AI is creating an impact! A few eye-opening insights which she has shared in this interview include:
- The impactful use of machine learning algorithms to tackle climate change
- The various data sources and processes involved in making data usable for machine learning and AI
- The rising concern of carbon emissions and how Climate Change AI is rising to the challenge!
Give the interview a read to have a fun and engaging experience!
Now is the time to act, and I encourage everyone to figure out how they can best leverage their unique skills and positions in society in order to tackle climate change.
– Priya Donti, Co-Founder & Chair, Climate Change AI
What is the main mission of your organization and how has AI played a vital role in shaping the mission?
Climate Change AI’s mission is to catalyze impactful work at the intersection of climate change and machine learning. In particular, as detailed in our report “Tackling Climate Change with Machine Learning,” machine learning can support or accelerate existing climate change strategies across many different sectors in order to help reduce greenhouse gas emissions and adapt to the effects of a changing climate.
At the same time, machine learning is not a silver bullet, and can also be used in ways that increase greenhouse gas emissions or exacerbate societal inequities. Our goal is to facilitate the impactful use of machine learning to tackle climate change by alleviating bottlenecks to doing work in this area, and by raising awareness about machine learning’s strengths, limitations, and potential side effects in the context of climate change applications.
What is the fundamental change, either economic or political, required to drive AI as an effective response against climate change?
While there is no one “fix-all” change, one fundamental challenge is ensuring a pathway to deployment for impactful applications of machine learning. For example, potential deployment partners such as city governments or power system operators often need new or different kinds of funding, expertise, and capacity than those readily available today in order to deploy AI projects.
Many climate change applications are not monetizable under current markets, which makes it difficult to employ these applications at scale. Additionally, a great deal of deployment-relevant data is often unavailable or hard to access. In some sense, some of the most-needed policy and economic changes here are broadly applicable, as opposed to being completely specific to AI. For instance, strategies such as setting aggressive decarbonization targets, mobilizing funding and talent to sectors where it is needed, and creating appropriate market incentives can create an ecosystem that drives effective climate action in general — including those actions that involve AI.
On the data side, public entities could play a major role in creating data ecosystems and otherwise encouraging the sharing of data relevant to implementing climate change strategies, though these efforts should be particularly mindful to mitigate any privacy concerns that may arise.
In general, how much change is AI capable of bringing about when it comes to climate change? How is your organization or project helping to bring about this change?
AI and machine learning can contribute to climate change strategies across many different sectors and areas by helping analyze data or optimize systems at scale. These uses can manifest themselves through applications as varied as inferring global greenhouse gas emissions from satellite imagery to inform policy, optimizing global supply chains to increase their efficiency, accelerating power system models to enable the use of time-varying renewable energy, and forecasting extreme events to inform disaster response efforts.
Our organization seeks to facilitate these impactful uses of machine learning by organizing programs and events aimed at providing education and infrastructure, building a diverse and multi-disciplinary community working in this area, and advancing discourse about important considerations surrounding research and deployment.
One of the biggest concerns of today is the rising carbon emissions from training AI & Machine Learning models itself. How do you see this tackled in the long run?
There are unfortunately a number of ways in which AI and machine learning may contribute to increasing greenhouse gas emissions. For instance, large machine learning models can be energy-intensive to run, and if this energy is provided by fossil fuels, then this comes with emissions impacts. Machine learning has also been used for applications such as optimizing oil and gas exploration, or to otherwise increase the competitiveness of emitting sectors.
In the long run, we will need a mix of policy and incentives that take a holistic approach to addressing the impacts of machine learning on climate change, both due to its energy use and through the applications for which it is used. Some examples include transparency and reporting standards around machine learning applications, and an explicit consideration of climate change impacts in countries’ national AI strategies.
It is critical that machine learning practitioners consider the context and use cases around any datasets that are available
– Priya Donti, Co-Founder & Chair, Climate Change AI
One of the biggest concerns when it comes to climate change is the lack of access to information and transparent data. How in your organization’s journey to using AI to tackle climate change are you getting over this barrier?
The conversation around data is an interesting one. On the one hand, there are many public data sources — such as satellite imagery and public power system data — that machine learning applications can employ. On the other hand, a lot of relevant data is often distributed, disorganized, and private, and often not in a format that is easy for machine learning algorithms to use.
Our organization is trying to address this data challenge by consolidating information about relevant public data sources where they exist, and funding the creation of research-ready datasets where there are gaps. There are also a number of other excellent initiatives — such as Climate TRACE, the Global Energy Data Commons, Icebreaker One, and the Public Utility Data Liberation project, to name a few — that are trying to tackle different aspects of the data challenge.
However, one common fallacy that I see often arise in the discourse about data is the assumption that if the data is public and “clean,” then the path to deploying projects is clear. In reality, that is not the case. It is critical that machine learning practitioners consider the context and use cases around any datasets that are available, and co-develop projects with relevant stakeholders from the get-go in order to ensure that these projects will actually be impactful. To facilitate this co-development, our organization aims to build bridges between machine learning practitioners and relevant stakeholders by providing venues for them to network and share ideas.
Do you view AI as the magic potion to tackle climate change?
No! In the end, AI is just one tool in a broader toolkit that can be used to support or accelerate existing climate change strategies. Climate change is a multifaceted issue, and addressing it will require many kinds of approaches applied across many different sectors. Now is the time to act, and I encourage everyone to figure out how they can best leverage their unique skills and positions in society in order to tackle climate change.
Has the current pandemic affected the organization goals in any manner? What are the next steps and milestones for your organization?
While COVID-19 has created a temporary dip in emissions, emissions are expected to rebound and potentially even increase after the pandemic, underscoring the importance of continuing to take strong and rapid action on climate change.
Our organization is committed to continuing to work to address the climate crisis, and is currently building out additional educational materials and (virtual) programs to spur impactful work in climate change and machine learning.
To stay tuned about our activities and learn more about how you can plug in, we encourage you to check out our website and sign up for our newsletter at www.climatechange.ai.