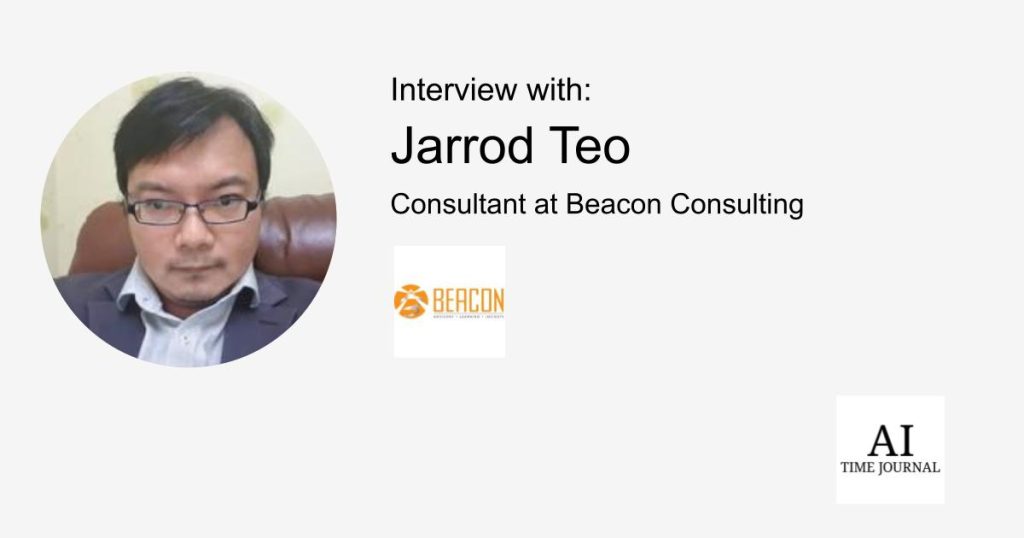
In this interview, I had the pleasure to converse with Jarrod Teo, a seasoned consultant data science leader, to delve into the intersection of data science and business strategy. Jarrod shares insights from his journey developing AI tools that propel business valuations, tackle data acquisition hurdles, and harness the power of large language models in commerce. His experiences offer a roadmap for integrating data science into business with a focus on practical application and adapting to economic uncertainties. Join us as we explore how these innovations and strategies are shaping the future of e-commerce and beyond, providing a glimpse into the skills and approaches essential for today’s data scientists.
Can you describe the development of your data science product that significantly contributed to a company valuation of $13.4 million? Certainly. The genesis of this product was a request from a merchant who lacked data to understand market trends in the US and China. Despite her company being more traditional, she sought to expand into new markets. This led to the creation of a blueprint for a product that could analyze market trends without relying on internal company data. This idea evolved into what we called ‘Ecommerce Watch,’ a tool enabling merchants to gather information from various sources and visualize it on a dashboard. This innovation allowed for insights into production trends and customer preferences in different countries, ultimately leading to the successful valuation of the company.
How did you overcome the challenges of acquiring data from external sources? The key was leveraging a diverse set of data, including numeric, text, image, and video data. We collaborated with a colleague skilled in text analysis, which was crucial for processing vast amounts of textual data from different languages. This approach allowed us to provide real-time, actionable insights for merchants, significantly enhancing their decision-making processes.
Reflecting on your journey of creating 15 Data Science products, what was a significant challenge you faced? A notable challenge was the impact of COVID-19. As the pandemic emerged, we needed to test and adapt our models to the changing economic landscape. We developed a product to measure model performance in these new conditions, ensuring adaptability even in unpredictable scenarios like COVID-19. Another challenge was meeting specific client requirements, such as making our products accessible and user-friendly on different devices, including mobile platforms.
As Secretary General of the Singapore Chamber of Commerce and Industry, how has your data science background influenced your role? My data science background has been instrumental in translating complex theories into practical business applications. It’s about understanding and communicating the value of data-driven decision-making in a business context. This approach has helped me bridge the gap between technical and business perspectives, fostering more efficient and effective strategies for commerce and industry.
From your perspective, what advice would you give to businesses integrating data science into their operations? Businesses should prioritize practical applications of data science and not focus excessively on infrastructure. Start with the data and build models that can be tested and scaled effectively. It’s crucial to plan for how these models will eventually integrate with online platforms and contribute to business goals like customer targeting and product recommendation.
How do you see large language models (LLMs) transforming the data science landscape, particularly in E-commerce and retail? LLMs are set to revolutionize E-commerce and retail by enhancing personalized shopping experiences. We’re moving towards a future where virtual assistants, powered by comprehensive data analysis, will provide tailored product recommendations. This evolution will lead to more efficient and engaging customer interactions in both physical and online retail environments.
Based on your extensive experience, what key skills are essential for modern data scientists? Beyond coding and tool proficiency, data scientists must understand and apply statistical methodologies and machine learning models practically. They should also be able to communicate these concepts to non-technical stakeholders and plan strategic blueprints for leveraging data in business contexts.
Considering current economic uncertainties, how should machine learning strategies evolve? In times of economic change, the key to successful machine learning deployment is a solid foundation in data handling and model testing. By ensuring thorough preparation and adaptability, data scientists can minimize the risk of model failure, even in unpredictable economic climates. This approach allows for continued relevance and effectiveness of machine learning applications, regardless of market fluctuations.