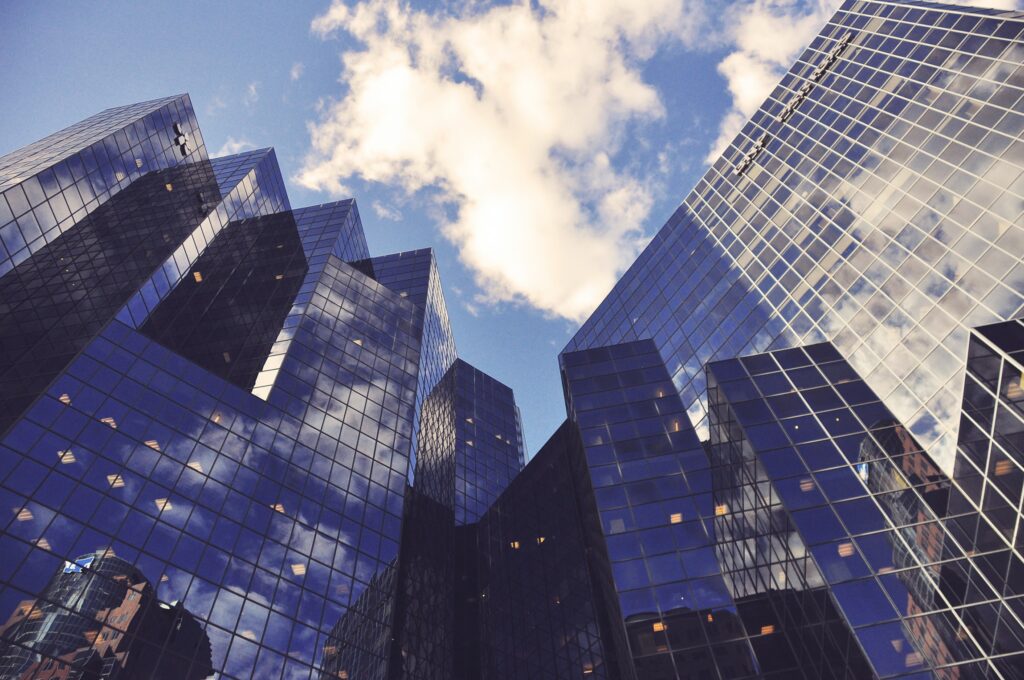
Banks are a basic part of economic growth. Banks have credit risk like other businesses in the financial sector. Foreseeing credit risk is the main area of worry for the banking sector in the majority of the nations around the globe. Credit risk emerges when borrower neglects to pay the money obtained. Lender utilised credit agencies for consumer credit history, anyway fast deviations in consumer behaviour and economic situations have made the credit department information untrustworthy for consumer reliability.
(Khandani et al., 2010) approvals that there are open doors for growth and risks in the customer loaning industry. The credit risk model is reliant on algorithms which produce creditworthiness score of the person.
(Härle et al.,2015) foreseen that customer expectation are different because of extraordinary changes in technology which prompted huge changes in the banking system. The banks can augment their revenue by focusing on the new technology-savvy young generation, which is growing in developed and developing nations. Banks must upgrade their process on customer experience for technology sagacious customers by the quick response on the result of the credit application. Also, Banks ought to upgrade system which can make a quick decision, seamless experience to their clients and evaluate applicant risk with no human intervention. Hence, banks can just endure and grow by distinguishing risk ahead of time and decrease in investment in bad loans.
Credit assessment is the process wherein the relationship is worked between features of new customers and old customers. Banks are applying data mining techniques to estimate the future behaviour of customers to decrease frauds and classify loan candidates with a high likelihood to repay. Thus, the victory of traditional financial institutions and peer to peer lenders depend on the solid predictive model for anticipating bad customer. As indicated by (White, 2017) Zest Finance and Ford Motors are utilizing Classification algorithm in lending loans.
(Yu, noDate) claims peer to peer lenders are more exposed to risk as compared to banks as they do not have enough information about the borrower. It is very significant for them to foresee the creditworthiness of the individual. Banks get information from the credit agency on the risk profile of the borrower. Along these lines, the machine learning algorithm is indispensable to find the likelihood of the defaulter. Likewise, (Han et al., 2018) characterize new banks use social media data from Facebook, LinkedIn, Twitter for anticipating individual credibility. Besides, (Sudhakar, 2016) explains data mining techniques supports in classification and forecast of appropriate features that determine the limit of the individual to pay off the loan.
(Hosmer at el., 1989) proposed Logistic regression (LR) is a commonly used algorithm in which the likelihood of an outcome is connected to a set of the conceivable independent variable.
Logistic regression is a linear algorithm with a non-linear change on the output. It assumes a linear relationship between the input variables with the output. Logistic regression assumes no error in the dependent variable consider expelling outliers and conceivably misclassified cases from your training data.
Logistic regression is named for the function utilized at the centre of the method; the logistic function called sigmoid function. It is an S-shaped that can take any real value number and map it in the range of 0 and 1.
Logistic regression is a linear method, but the predictions are changed utilising the logistic function. The effect of this is that we can no longer comprehend the predictions as a linear combination of the inputs.
Key points should be taken into consideration while building the Logistics Regression Model. Firstly, independent variables in the logistic regression should not have high multicollinearity in between them which means independent variables should not have a high correlation between them. It very well may be checked through Variation Inflation Factors (VIF) value and VIF value greater than 10 is a concern (Chen et al.,2018). Furthermore, an alternative combination of predictor variables to response variables. The combination of predictor variables with the lowest residual deviance should be used to make the model better. The Akaike Information Criterion (AIC) can be used to compare different models of logistic regression for the goodness of fit. The model with the lowest possible AIC is the most excellent model. In addition, stepwise logistic regression techniques are used to build credit scoring models in the bank. It yields the most log-likelihood with the least number of parameters for the model (Liou et al., 2018).
Fisher score supports in finding how the model was estimated. The model tries to improve by using different estimates. Fisher score iteration supports in informing us how many iteration algorithms run to get the best model (Jaakkola at al., 1999). On the other hand, confidence intervals aids in the estimation of logistic regression coefficients (Hosmer et al., 1992).
The coefficients of the logistic regression must be evaluated from your training data. This is finished utilising maximum-likelihood estimation. It is a normal learning algorithm utilised by various machine learning algorithms, in spite of the fact that it makes assumptions about the distribution of your data.
With increased competition and risk in the lending industry as well as reduced margin, credit Industry wants to diminish credit risk based on data. This investigation will help with reinforcing assessing credit risk procedure in the financial institution.
Bibliography
- Hosmer, D.W. and Lemeshow, S., 1992. Confidence interval estimation of interaction. Epidemiology, pp.452-456.
- Hosmer, D.W., Jovanovic, B. and Lemeshow, S., 1989. Best subsets logistic regression. Biometrics, pp.1265-1270.
- Jaakkola, T. and Haussler, D., 1999. Exploiting generative models in discriminative classifiers. In Advances in neural information processing systems (pp. 487-493).
- Liou, J.W., Liou, M., Cheng, P.E. and Lin, C.C., 2018. Modelling Interaction Effects in Logistic Regression: Information Analysis. arXiv preprint arXiv:1801.01003.
- Chen, X., Huang, L., Xie, D. and Zhao, Q., 2018. EGBMMDA: Extreme Gradient Boosting Machine for MiRNA-Disease Association prediction. Cell death & disease, 9(1), p.3.
- Hosmer, D.W., Jovanovic, B. and Lemeshow, S., 1989. Best subsets logistic regression. Biometrics, pp.1265-1270.
- Han, J.T., Chen, Q., Liu, J.G., Luo, X.L. and Fan, W., 2018. The persuasion of borrowers’ voluntary information in a peer to peer lending: An empirical study based on the elaboration likelihood model. Computers in Human Behaviour, 78, pp.200-214.
- Sudhakar, M., Reddy, C.V.K., 2016. Two-Step Credit Risk Assessment Model for Retail Bank Loan Applications Using Decision Tree Data Mining Technique. International Journal of Advanced Research in Computer Engineering & Technology (IJARCET), 5(3), pp.705-718.
- Yu, X., (noDate). Machine Learning Application in Online Leading Credit Risk Prediction.
- White, W., 2017. Ford to Use Machine Learning for Credit Approvals. Available from http://www.nasdaq.com/article/ford-to-use-machine-learning-for-credit-approvals-cm837459 [Accessed on 11th February 2018].
- Härle, P., Havas, A., Kremer, A., Rona, D. and Samandari, H., 2015. The future of bank risk management. London, UK: McKinsey & Company.
- Khandani, A.E., Kim, A.J. and Lo, A.W., 2010. Consumer credit-risk models via machine-learning algorithms. Journal of Banking & Finance, 34(11), pp.2767-2787.
Image Credits
Featured Image: Unsplash