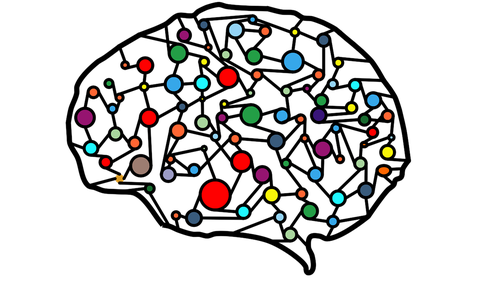
The symbiosis between machine learning and Master Data Management may not be readily apparent. After all, the former delivers targeted analytics for cognitive computing, whereas the latter consolidates aspects of data governance, data quality, and data modeling. Upon initial consideration, they appear to be from two distinct regions of the data management landscape.
However, many of the standards MDM enforces for governance and data quality are critically important for devising credible machine learning models. Conversely, the automation machine learning furnishes is tremendously useful for expediting numerous tasks required to make MDM actually work.
According to Profisee Product Marketing VP Martin Boyd, “If the data is complete and consistent, then you can use machine learning much more effectively. A lot of [organizations] have recently quoted that as a reason why they’re adopting MDM.” Alternatively, when MDM solutions are fortified with certain machine learning technologies, they considerably improve their means of providing the benefits Boyd referenced.
Thus, MDM assists machine learning with the data staging required to make models perform well, while machine learning aids MDM by accelerating time to value and expanding the sway of personnel necessary to ready the data for those models, including data modelers, data stewards, and compliance officers.
Data Preparation
Enterprise deployments of machine learning hinge on the data preparation necessary to build accurate models. Oftentimes, such data engineering—which involves adhering data to a single data model, cleansing data, and aligning varying definitions and terms—can considerably slow or prevent such endeavors from coming to fruition. By definition, MDM standardizes these aspects of training data so data scientists can tailor advanced analytics models to solve business problems.
“One of the big reasons to implement MDM as part of digital transformation initiatives is, when you’re thinking about that initiative, you’re thinking about what insight can I get from the data,” Boyd reflected. “You’re nearly always thinking about using machine learning to drive those insights. But, if the data is not complete, consistent, and accurate, then any machine learning algorithm you apply to it will not be able to operate properly.”
Data Quality
MDM provides these necessities and others across sources systems, repositories, and toolsets to reinforce data quality. It does so with numerous techniques, including data quality rules for transforming data so they conform to predefined conventions stipulated by organizations.
Boyd mentioned a use case in which Domino’s Pizza relied on MDM to curate data for machine learning by “standardizing and merging customer information to get the best understanding of a customer’s usage and preferences of their online ordering. They used that to drive machine learning algorithms to identify those customer preferences and then perhaps give customized offers, either real-time during the ordering process or in the form of coupons or discounts, to encourage them to come back.”
Ensuring quality of data is indispensable to the success of those or any other machine learning algorithms. For such an algorithm, any dearth of data quality “might completely destroy its ability to have a meaningful insight,” Boyd warned. “Or, at a minimum it’s going to impair it and make it less impactful.”
Mastering Data
Machine learning plays a vital role in some of MDM’s most fundamental functionality. Regardless of the domain organizations deploy it for (which frequently includes customer, product, supply chain, and more), the capability to match and merge records—a significant amount of which is assistive to data quality—is crucial for success in this branch of data management.
Supervised learning techniques and others are able to make these responsibilities easier for organizations by delivering much needed automation for certain aspects of these tasks. “We’ve used machine learning techniques in our matching for a long time,” Boyd commented. “It’s a machine learning algorithm that’s at the core that does the matching and merging of records.”
Machine learning’s advanced pattern recognition capability is well established for detecting aberrations or outliers that have critical business value—such as pinpointing differences in user behavior that may impact cyber security analytics, for example. Concentrating these capabilities on the myriad aspects of data stewardship that are foundational to MDM is another area in which cognitive computing improves master data. “We are in the process now of developing machine learning capabilities that will assist data stewards in identifying data anomalies and resolving them,” Boyd remarked.
Mutually Beneficial
MDM and machine learning work well together in what’s effectively a virtuous cycle. MDM standards for data quality, data modeling, and record merging/matching are some of the better ways to stage data for machine learning models with complete, recent, accurate data.
Additionally, machine learning is employed by MDM hubs to reduce the time to value in working with these solutions to reinforce aspects of data stewardship, data governance, metadata management, and more. Each of these technologies is a natural fit for furthering the aims of the other.
Featured Image: NeedPix