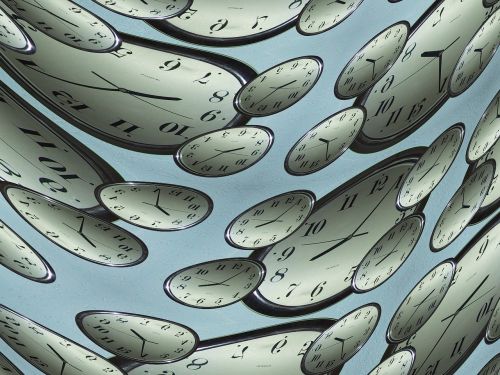
The assessment of data systems as they actually are, as opposed to what they were previously or are predicted to be, is arguably the paragon of contemporary analytics. Coupling this capability with machine learning enables organizations to not just make the right business decisions, but to capitalize on them in real-time.
Achieving this ideal requires more than streaming platforms such as Kafka. The tooling doesn’t just involve the right data science models, networking capabilities, or cloud providers.
At some point, it comes down to the performance of the underlying database, particularly in regards to concurrency and scale. Aerospike CPO Lenley Hensarling described that performance as “sub-node second latency with massively high throughputs. Meaning that, not only [is there] predictable performance of the sub-node second response, but [it applies to] hundreds of thousands of transactions going on while supporting millions of transactions per second.”
Solutions processing data at that scale and speed are not only optimal for gathering relevant training data for machine learning models, but also for deploying them for low latent action in the Internet of Things, edge computing, and numerous other real-time analytics use cases.
“In the context of any decision, it’s better to have a more complete, up-to-date picture of the context: all the data applicable to any given decision,” Hensarling explained. “This means you have to ingest tremendous amounts of data…and apply that in the context of the decision.”
Core and Edge Computing
Oftentimes, the first way data at this scale aids the enterprise decision-making process is via the creation of machine learning models to solve problems like fraud detection, for example. The scalability of the type of database Hensarling referenced is primed for simply amassing the quantities of training data necessary for this undertaking. Such data is also pivotal for feature generation and refining models before putting them in production. “In these core databases, which oftentimes might be up to petabytes in size, we allow people to do a lot of machine learning, a lot of analytics against that data, and create features as an AI/ML thing,” Hensarling revealed.
This use case also typifies the juxtaposition of centralized computing versus edge computing, while attesting to the correlation between the two. For fraud detection, those machine learning models may actually be implemented remotely at point-of-sale systems. “You can push those features to the edge, in our database, where they can be used in analytics that are real-time analytics,” Hensarling commented. “I have to apply this and make a decision: is this fraud or not? Is this a situation in which I should do this trade or not?”
Low Latency
The synthesis of machine learning and real-time analytics supports a number of use cases across verticals, including aspects of fintech, martech, insurtech, and others. Recommendation engines are the quintessential example of this pairing. In adtech and other areas of e-commerce, organizations only have fleeting seconds (if that) to place content in front of buyers to capitalize on up-selling and cross-selling opportunities. This use case revolves around recent purchases, on-screen clicks, and the length of time in which users look at various pages, products, and services.
It also may incorporate additional data sources from CRMs and other systems with information about customers’ purchasing habits. “If you’ve got a recommendation engine, you’re applying that to hey, here are things at the bottom of your screen when you’re buying something,” Hensarling remarked. “Do you also want to buy this? You’re serving that up and making a decision about what to put there in real-time.” Substantial revenues are generated from relying on machine learning models to surface the proper content to entice customers into additional purchases.
However, organizations can also improve these models—in addition to their real-time decision-making processes—by scrutinizing how successful recommendations were. Doing so requires retrospective analysis of what’s now historical data to denote areas for improvement. “People want to go back and say, how efficacious was that?” Hensarling pointed out. “Did I really do well with what was put out there? And so, that’s a different kind of analytics that’s not so much real-time, but goes back into all that collected data.”
Next Best Action
The possibilities for acting on real-time event data with accurate machine learning predictions are just as viable for facets of risk assessment (like network security) as they are for profitability. Databases that support low latent analytics for such timely action are essential for these endeavors, providing what Hensarling called “real-time context” for the best decisions, and actions.
Featured Image: NeedPix