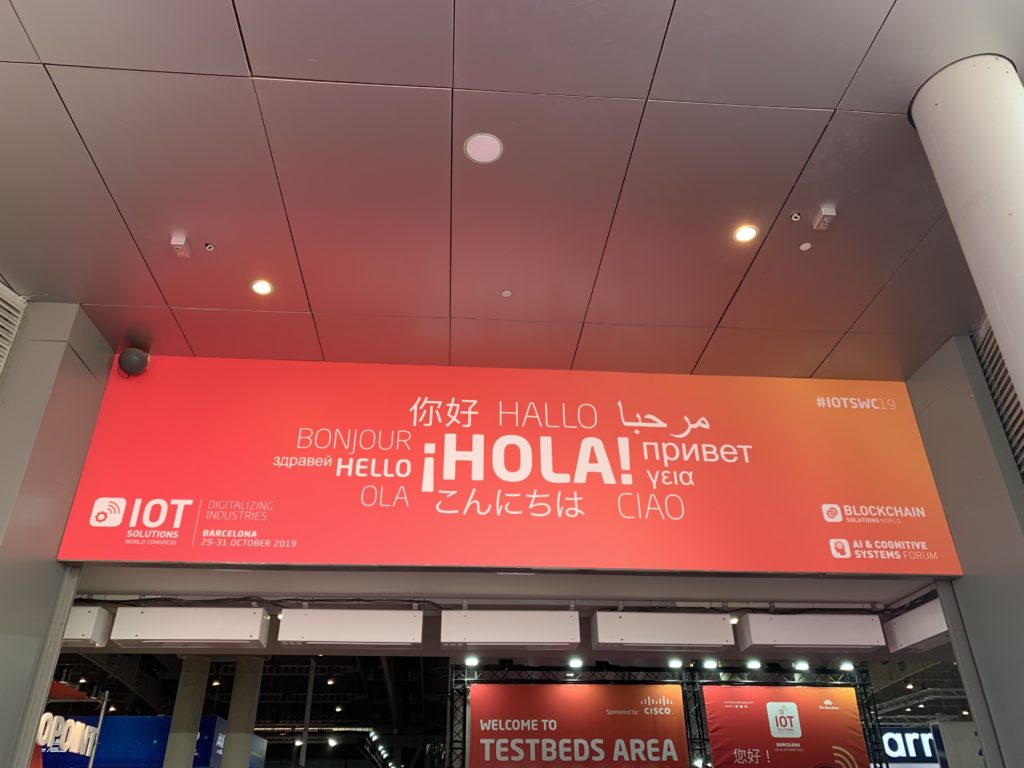
Two weeks ago I visited the IoT WorldCongress 2019, which took place from the 28th to the 31st of October in Barcelona. As my first AI related congress I was going to attend, I was very excited about it. I am one of the, every day less so, few professionals standing just in the intersection between AI and Healthcare. In other words, my job is to apply the power of AI to Healthcare data so patients get better experiences and results during treatment. Being that my context, it was obvious that the IoT WorldCongress, with specific tracks for Healthcare and the AI & Cognitive Systems Forum between others, would be a very interesting event to go to.
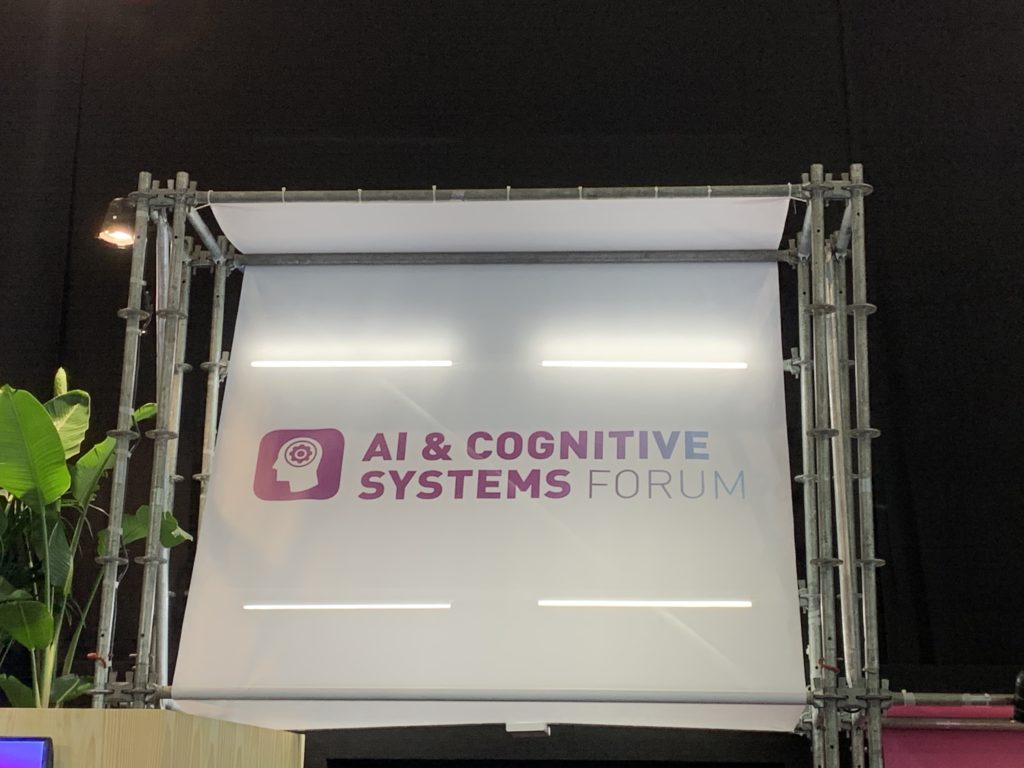
And as expected, I was not let down by the contents of the sessions. It will not be possible in this article to explain in detail all of the cool ideas exposed during the congress, so instead I will highlight the top 3 takeaways that stuck with me about the present and future of AI and IoT regarding Healthcare.
The promise of the “Quantified Thyself”
Nowadays almost everybody uses devices connected in some way to the net, and between those devices the wearables hold a highly powerful place. Controlling constantly our activity level, our sleeping time and quality, and even in some cases our heart ratio, it is self-evident that they are a precious source of data about our health.
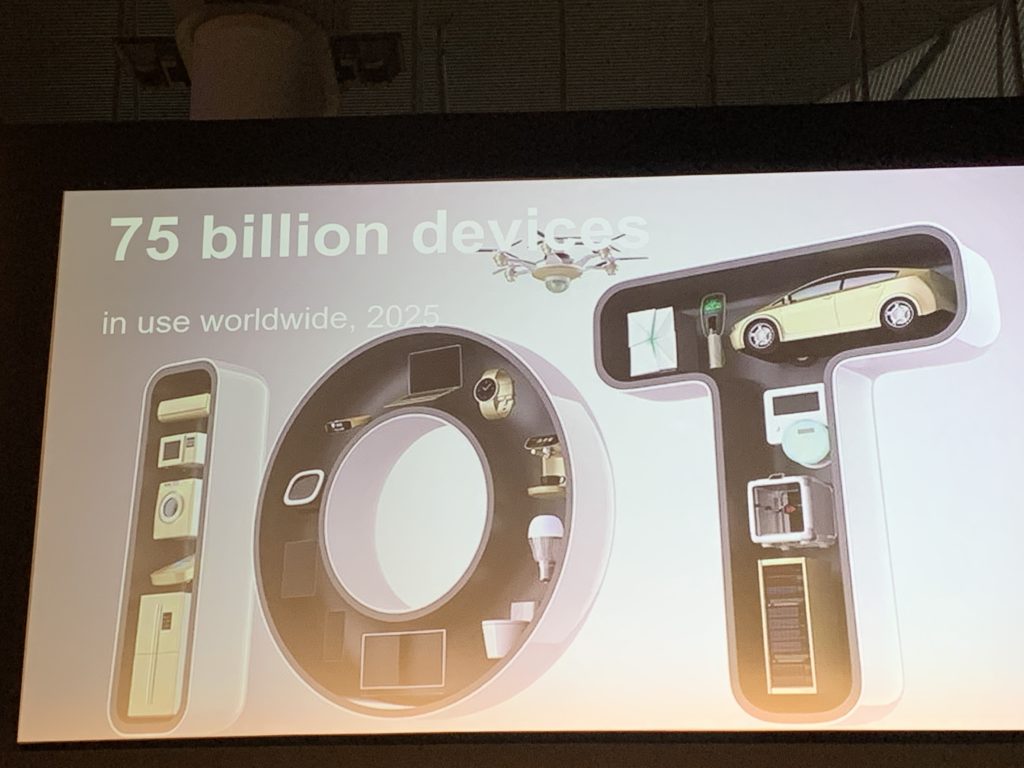
To make good use of this wellspring of data, several speakers proposed app based systems to implement a remote follow up with the patients. From the patient’s perspective, this would open a fast and easily accessible point of contact to their doctor, while for the medical expert it would render a continuous report of the patient’s evolution.
Specifically, the collaboration between the corporation HealthITcare and Barcelona’s Vall d’Hebrón public Hospital made a strong point in this regard. Focused on patients from the mental health department, this specific implementation makes for an excellent example of how AI and IoT can improve quality of life for patients and families.
A mentally challenged patient would normally only go to the doctor during or after a crisis, which renders the treatment to only be able to deal with the aftermath of the current drop on the health of the patient. As showed with this collaboration, the 24/7 monitorization of the patient’s vitals via wearables, and of the digital phenotype (info gathered about their behaviour in their activity on the phone) could help to even prevent such crisis. This info gathered by their own designed app is anonymized, sent to their cloud and fed to an ML algorithm trained to generate a report of the patient’s present state of health. The clinician receives this already curated report and can take immediate action if necessary.
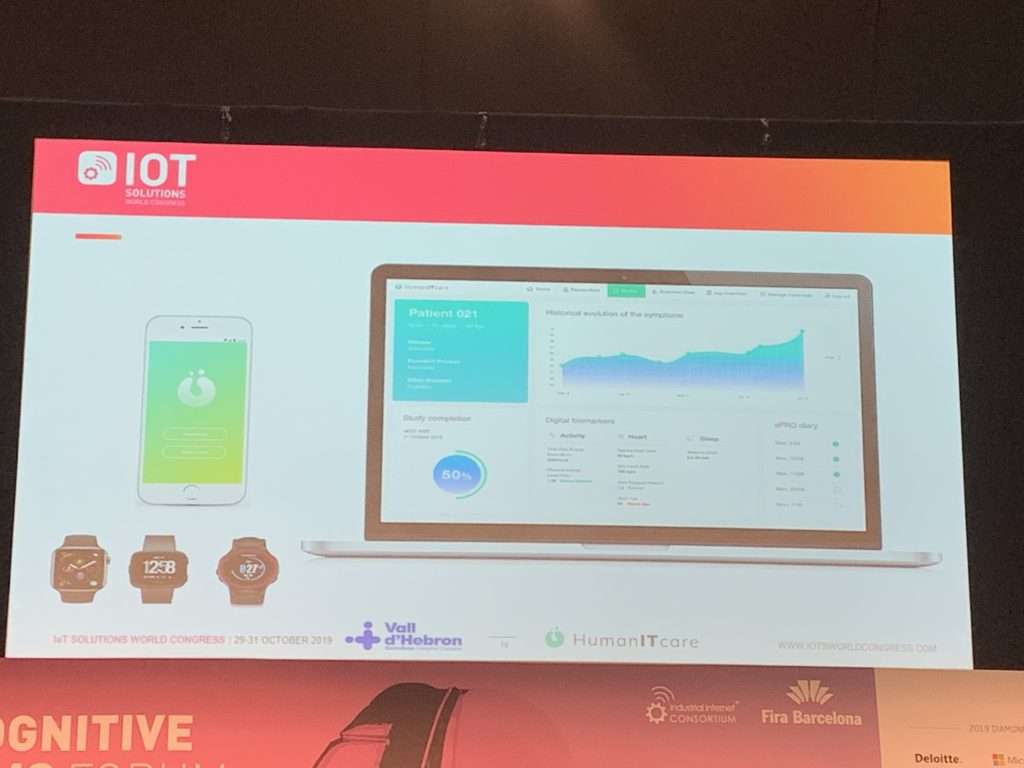
Such implementations of IoT in combination with AI already happening show us the great potentiality of this alliance in benefit of a new modality of medicine, with personalization and prevention (more than reaction) as its new goals.
Getting back the power to the user over their own data
If you are a data scientist you know that the struggle of getting the data you need for your investigation is very real. And AI and ML are nothing without data.
In healthcare, the patient is the source of this data, and traditionally it is gathered in hospitals/clinics where they are tended to. This data is necessarily provided by the patient so they can be cared for, and maybe later in time can be used by investigators in the medical centre to make observational studies or train a predictive model. Access to this information, in any case, is regulated by law to protect the privacy of the patients.
Nowadays though, with so much information about it or health being introduced to our phone’s apps (via wearables or manually) there is a new input of healthcare data other than the traditional one. Although most of the time the user doesn’t think about it, this data in some cases can be and is sold to third parties to make their own studies. This is nothing new, and events such as the uproar around the well-known Facebook incident show the importance of user privacy and the not so good use some corporation can make of our data. The difference here is that we are speaking about health data, which can be even more delicate, and it is not as well protected by law as the one that is generated in a clinic environment.
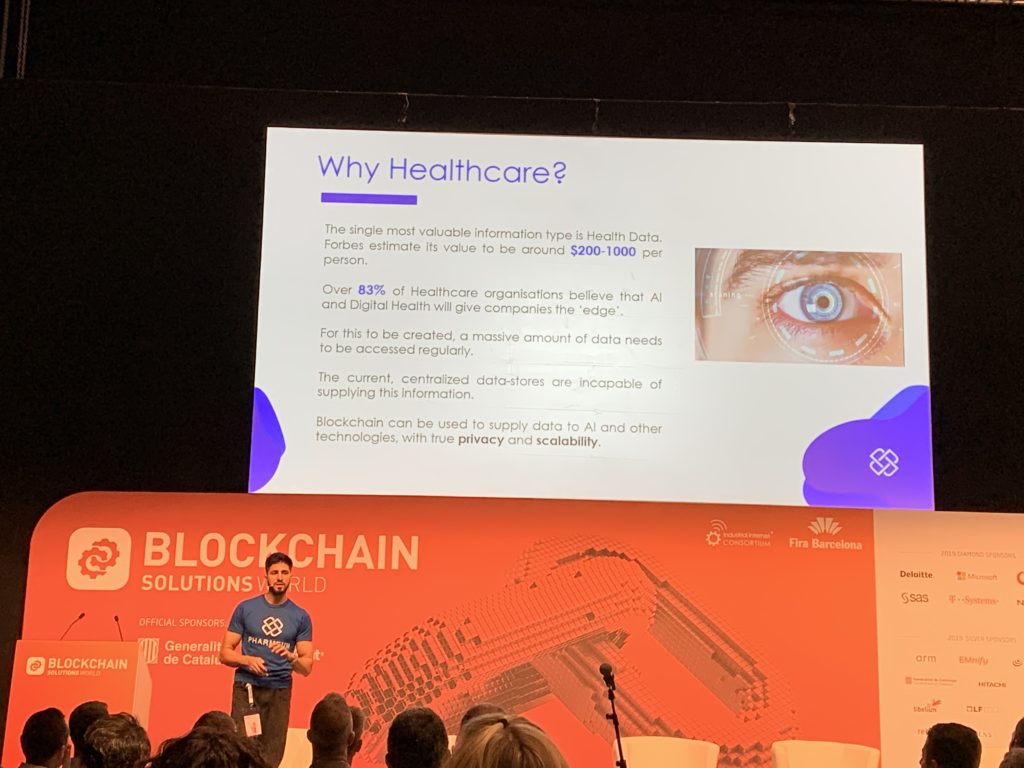
In this context, the speech by Zain Rana, CEO of Pharmeum, made, in my opinion, a very valid point. As I mentioned earlier, sometimes your data is the source of revenue for someone and you don’t get a penny for it. So, why not sell it willingly and knowingly and get something in exchange? That is what Pharmeum offers, and in my opinion is genius. An app based on private Blockchain provides a platform for both users and researchers to connect. The users have the power over their own data and get PHRM coins every time app developers use it. This idea is, then, one valid way to solve two problems in one go: easy access to data by researchers, and getting back agency over own health data for the user.
With great power comes great responsibility
This quote by beloved Stan Lee seemed to be a common mantra to every speaker giving a speech about ethics in AI. And it is just spot on. It is common knowledge around data scientists that one big hindrance in developing an algorithm is in the bias that our dataset can have. And as a chief research scientist from Accenture Labs Edy Liongosari said, AI can amplify human biases.
Sometimes it can be very difficult to correct those biases, as it was discussed in the panel that followed Mr Liongosari’s speech about responsible AI. But it is actually really important, especially in the field of Healthcare, that we try as much as we can to avoid it.
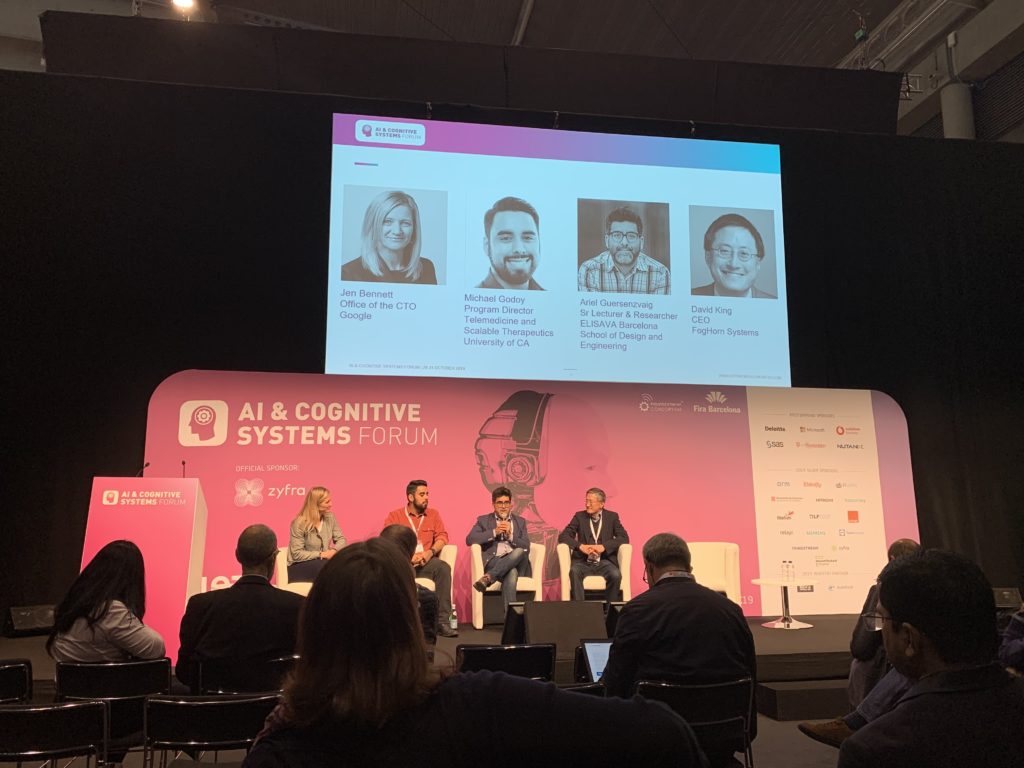
One very good example of why we should do so is explored in the recent study published in Science this October by researchers at the University of California, Berkeley. It showed that an algorithm used by many hospitals in the US was heavily biased, discriminating against black people in the event of being referred to more intensive healthcare programs.
Bias in the dataset, amplified by the algorithm, which led to one sector of the population not to receive adequate treatment for their illness. This is simply unacceptable. And even if this specific algorithm is being revised to correct the bias, we, as data scientists focused in Healthcare, must be always alert and careful to not create this kind of situations.