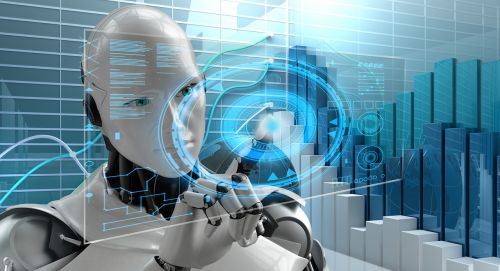
Depending on whom one asks, Artificial Intelligence’s utility for time-honored data management problems involving data integration and data architecture has been highly exaggerated. In this article, we will review Artificial Intelligence in data integration.
According to Gartner’s definition of a data fabric (which unites enterprise data regardless of location to make them singularly accessible), AI techniques are central to performing the data integration that makes this architecture a reality. To the credit of the analyst firm, certain inference mechanisms pertaining to knowledge graph technologies can automate aspects of data integration.
However, according to Cambridge Semantics CTO Sean Martin, “This is 2023. Everybody wants AI, but the amount that it will actually do for you [for data integration], really, is still somewhat limited. It’s a magic crank; it doesn’t exist.”
Granted, there are non-statistical AI methods that can assist with facets of data integration. Numerous machine learning approaches are apposite for assembling information into knowledge graphs, which are useful for identifying relationships and points of commonality in data.
But, it appears that the capability to fully automate all aspects of data integration for enterprise-spanning architectures like a data fabric—or anything else—is not yet upon us.
Active Metadata
The general premise for utilizing AI to automate data integration relates to the tenet of active metadata, which Gartner has also been championing. Metadata assists with data integrations by providing lineage about previous successful integrations, which vendors or organizations can use in the future to optimize—and accelerate—future data integrations. The active metadata notion purports to extend such functionality to provide dynamic integrations based on AI technologies such as machine learning. This capability is said to have particular relevance for data fabrics.
“The whole notion of using AI to fully automatically use metadata to do data integration is overblown,” Martin stipulated. “That doesn’t really exist in reality. AI may be able to assist here and there, and it does, but it’s not like you can just say, ‘We’re going to use a data fabric and offload it into AI, and now our data integration issues are over.’”
Some of the ways AI helps with data integration is via semantic knowledge graphs. The standardized data models and taxonomies characterizing these constructs are ideal for evolving schema to account for new business requirements and data sources. Proper implementations are even able to facilitate a degree of interoperability between respective data systems. However, the real-time applicability of this approach for a data fabric or data mesh architecture is limited.
Knowledge Graphs
Because of the aforementioned semantic capabilities and mutable data models of standards-based knowledge graphs, these frameworks have become increasingly valuable to data integration activities for data fabrics and data meshes. Moreover, because of techniques involving Graph Neural Networks (GNNs) and neural graph databases, these applications are desirable for coupling statistical and non-statistical AI approaches. “AI is a continuum of things,” Martin commented. “You can do inferencing with AI. There’s reasoning, like OWL-based reasoning. But you can also do predictions. Link predictions are an example of AI that’s trying to figure out where things should be connected.”
Both GNNs and neural graph databases typify capabilities for using link predictions to understand how to connect the information in datasets, which can be helpful for understanding how to integrate datasets. Those connections are also practical for populating a knowledge graph of a particular domain, which in turn can be of service for data integrations. Additionally, entity resolution is a critical precursor for certain data integrations; knowledge graphs can enhance this practice. According to Martin, entity resolution is “where you’re trying to say that this entity in this dataset is the same entity in that dataset. Or, maybe they’re just related. Or, maybe it’s collapsing two entities together into one in your knowledge graph. There are many techniques that have been used for years for entity resolution. Now, some of those are increasingly using AI approaches.”
Not Quite There
With the recent sensation caused by Generative AI, virtually no one can dispute that this sundry of technologies and techniques is more influential than it’s ever been to the enterprise. However, AI’s worth for data integration, even for supporting contemporary architectures such as data mesh and data fabric, isn’t quite commensurate with its proficiency in generating images.
“The long and short of it is AI does not magically do the whole [data integration] job for you,” Martin reflected. “It’s still on the margin. I keep seeing that somehow, we’re going to use metadata with AI and do all this magic stuff. We’re just as much using the data as well as the metadata. But nonetheless, it’s still limited in what it can actually do for you.”