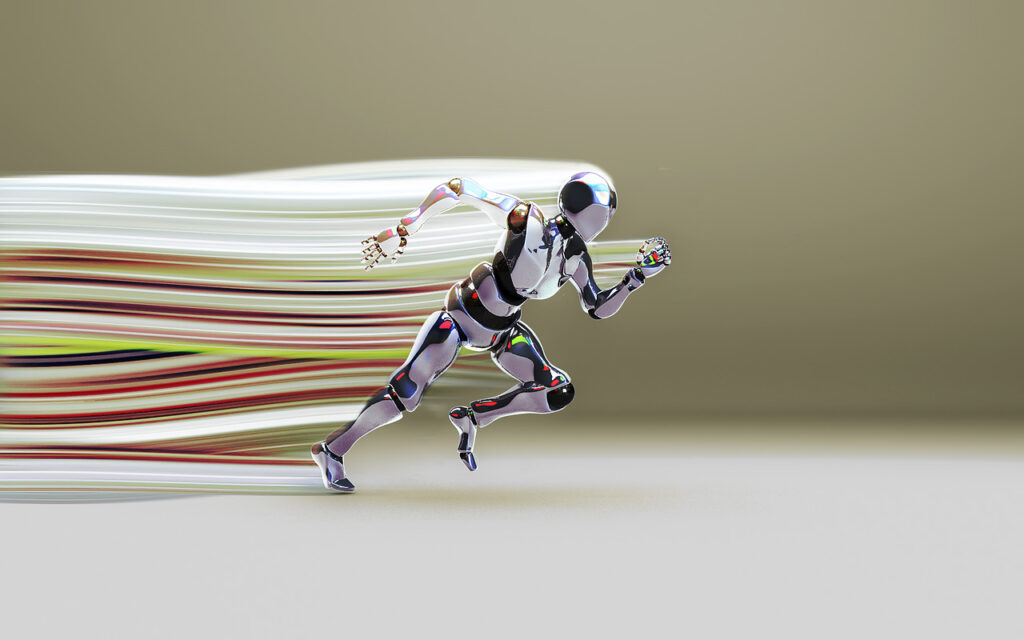
Several of the most advanced applications of Robotic Process Automation (RPA)—including employing bots to discover new automation processes or mimicking human employees with a digital workforce—hinge on deployments of computer vision, natural language technologies, and deep learning.
Consequently, the cloud is becoming the de facto environment for the fusion of RPA and the machine learning models required to produce tangible business results from these enhanced capabilities. According to Automation Anywhere SVP of Products and Engineering Abhijit Kakhandiki, the cloud is the ideal setting for pairing bots with statistical Artificial Intelligence for multiple reasons, including the ease and speed of deployment.
“One of the frictions in the RPA world was when you actually sign a deal, then you start procuring hardware and by the time you deploy, it might have been three months,” Kakhandiki mentioned. “Now in the cloud, you can sign up…and get going in the cloud that very day.”
The low latency of this paradigm also applies to individual use cases for leveraging RPA with dynamic cognitive computing models, including those involving a Cloud RPA. In this respect, cloud architecture is drastically improving the efficiency and efficacy of these critical computing capabilities.
Model Management, Updates
The lifecycle management of machine learning models involves continually updating them with the latest, most relevant data to ensure they’re properly functioning. Whether those models support a bot’s ability to observe a human’s screen and duplicate his actions for payroll, for example, or underpin analytics to Know Your Customer (KYC) in finance, the cloud is perfect since “what it does for AI is now we, as well as other vendors, have the capability to ingest tons and tons of data and even use cloud compute capacity to be able to update these models in increasing frequency,” Kakhandiki revealed.
The celerity at which data resources are accessible via the cloud underpins this advantage, which is highly significant for refining models in production. With this approach, “If you update an AI model every quarter, now you can do it every month or week, or even every day,” Kakhandiki disclosed. “That can actually affect your results immediately in terms of the decisions that you make and so on, because you have up-to-date trained AI models.” As a result, there are numerous organizations adopting cloud architecture for “business applications such as Anti-Money Laundering, continuous monitoring for procurement integrity, and model lifecycle management,” denoted SAS EVP and Chief Sales Officer Dave McDonald.
Peerless Scalability
The cloud is universally renowned for its horizontal scalability, which is ideal for synthesizing RPA with machine learning. Moreover, many of its boons for model management pertain to its scalability which supports the rapid inclusion and processing of enormous data quantities. This aspect of cloud architecture, in conjunction with its cheap storage, is one of the premier reasons why “technologies in the cloud such as APIs, microservices, elasticity, and other cloud services are all becoming table stakes for this business agility we’ve heard of,” McDonald reflected. The elastic benefits of cloud computing are invaluable to scaling for the demands of RPA deployments, RPA’s synthesis with AI, and AI itself.
“Not only does the cloud provide this intuitive consumer like experience with drag-and-drop simplicity and all of that, it also allows you to scale seamlessly because it’s elastic,” Kakhandiki remarked. “Whether you want one bot or you want thousands of bots, we can scale according to your demands…so that you don’t have any hardware to buy.” The cloud’s elasticity is also beneficial in healthcare for “developing innovative models to help with hospitals like Cleveland Clinic…for forecasting patient volume, bed capacity, and ventilator availability,” McDonald commented.
Configuring RPA and Machine Learning
Perhaps the final point of compulsion for deploying RPA and cognitive computing models in the cloud is the capability to couple these services with approaches involving the Application Programming Interfaces (APIs) McDonald referenced and other means. Organizations can readily access both RPA and advanced analytics models as Software as a Service (SaaS) offerings. One of the underlying boons of cloud architecture is that when organizations subscribe to an RPA service, “you can now seamlessly configure with any of these AI vendors in the cloud and send them data,” Kakhandiki noted. Thus, organizations can use data gathered by RPA to inform cognitive statistical models in this setting when “that AI model is running in the cloud it can actually ingest any amount of data, and I can update it with any frequency, without having to worry about adding hardware and all of that,” Kakhandiki continued. “You just kind of subscribe to it and everything else is taken care of.”
Cloud First
The coupling of RPA with advanced analytics will likely persist for some time to come. Creating, managing, deploying, and perfecting those models in the cloud will also likely continue as organizations rely on this architecture for its scalability and low overhead advantages. Leveraging this combination of technologies in the cloud works so well it could possibly become one of the more compelling drivers for adopting cloud architecture.
Image Credits
Featured Image: NeedPix