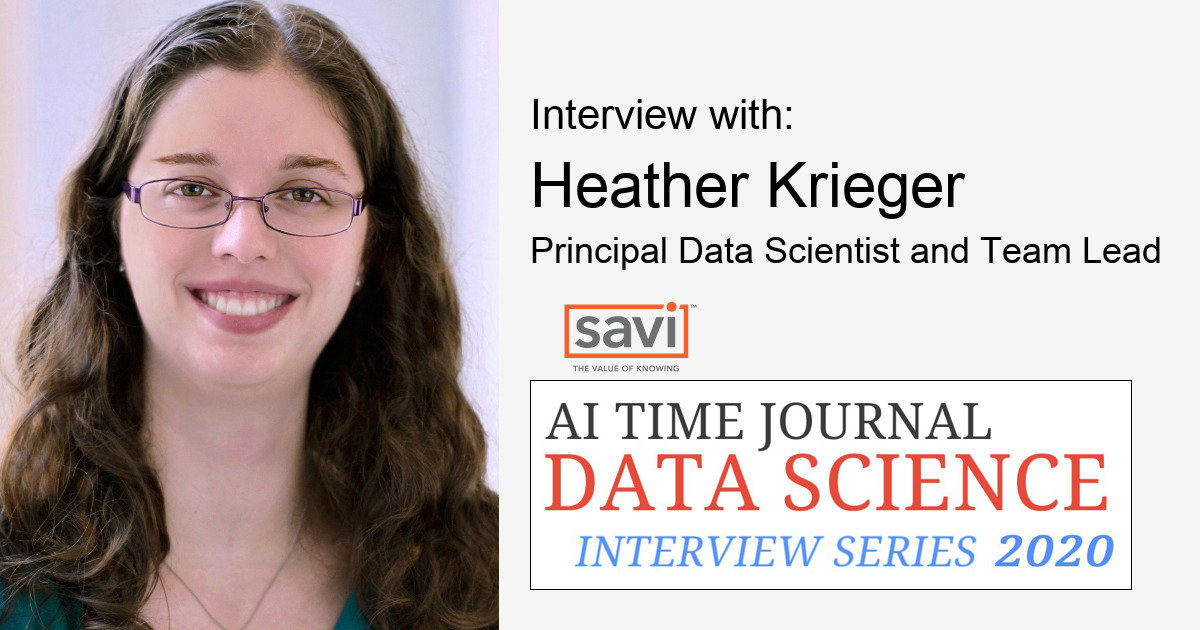
We thank Heather Krieger from Savi Technology for taking part in the part of the Data Science Interview Series 2020 and sharing her story of how she got into data science along with her views on ethics and data science and several insights and advices for beginner data scientists.
At what point did you realize that you wanted to pursue a career in data science, and how did you get into it?
I stumbled upon data science. I was in a PhD program and was starting to apply to post docs and academic jobs, but the market was/is rough, and I was trying to coordinate geographic locations with my spouse. An email soliciting applications for a data science fellowship/bootcamp popped up in my inbox and I decided to apply for it. Did a bit more reading into what data science is and what a data scientist does and decided it would be a great fit for my skills and what I wanted to do. Bonus that it made finding a job a lot easier.
How is data science used to create value in your current project(s)?
I work in supply chain technology. My team and I create algorithms to predict estimated times of arrival (ETAs) for global shipments across transportation modes and through stops like ports and customs. These algorithms detect anomalies in shipment behaviors and identify risky behaviors and locations for high-value goods.
Using data science in supply chain analytics helps companies answer key questions like “Where is my shipment and when will it arrive,” and be more efficient in their operations. Data science is emerging as a big differentiator in logistics, enhancing in-transit visibility making companies proactive rather than reactive.
The Savi team works closely with our customers to identify their specific supply chain issues and provide data-driven solutions that increase the efficiency and security of their operations while decreasing the cost of shipping and loss of goods. For example, one manufacturer we support was losing fragile product due to breakage in-transit. We were able to determine which carriers and methods of transport were resulting in significant loss. Our customer saved millions of dollars in product by moving their shipments to safer transportation modes.
What is one of the best investments that has propelled your data science career the most?
The bootcamp that I was accepted into and completed in person was fundamental in making my transition from academia to data science. I already knew a lot of statistics and research methods but had to learn the coding and big data wrangling practices. Learning in a group of 10 other students was invaluable. The program was free but did require moving to D.C. and did not cover living expenses. It was an intense 8 weeks but I learned a ton, met great colleagues who became good friends and sounding boards for problems, and was coached on getting jobs in data science.
What are the top challenges you currently face as a professional data scientist, and how do you go about tackling them?
Keeping up with all the new developments in the field is hard. I learn as I work through a problem so my main method of trying to keep up relates to looking for answers to current issues, errors, or questions. It is probably not the most efficient strategy, but it does ensure that I remember what I have come across.
I also learn a lot of new things from my colleagues whether they be on my team or at conferences. Hearing about how others are using data science to solve problems is always an eye-opening experience!
How important is the domain knowledge of the business/industry you’re in as a data scientist, and how did you acquire it?
Domain knowledge is crucial to data science. Without understanding the context of the data – how it is generated, why it’s collected, and under what constraints, etc. – you cannot understand the potential power and shortcomings of it. Additionally, without understanding the business use case for the data you cannot develop and answer questions that provide value. Lack of domain knowledge can lead to faulty results which can have pretty serious consequences.
What unusual or absurd thing do you practice or advocate for in your profession as a data scientist?
Sometimes the data you are given cannot answer the questions being asked or they do not produce the answer some might be hoping for and in those situations, you have to stand by your ethics.
Well I don’t think it is unusual or absurd but something that is not talked enough about in data science is ethics. You can train a model or wrangle your data to get almost any answer and your own knowledge and ethics are what maintain the quality, reliability, and validity of the work you produce. Everyone has heard the adage ‘garbage in, garbage out.’ Sometimes the data you are given cannot answer the questions being asked or they do not produce the answer some might be hoping for and in those situations, you have to stand by your ethics.
What inspires you about working in data science?
I love solving problems. The ability to work a problem out from beginning to end and see it put into practice is definitely what gets me out of bed and excited to go to work. I will always be amazed at what problems can be solved by combining big data in new ways and applying machine learning algorithms.
What advice would you give to someone who wants to get into data science today? What advice should they ignore?
The best data scientists I know continue to learn regardless of where they are in their careers. You cannot know everything about data science and, despite what many job postings make it seem like, none of us do. Data science can be used in so many contexts so learn about what interests you and look for jobs that compliment your knowledge, interests, and skills. With that said – don’t let lack of knowing something prevent you from going after data science whether it be a particular job, problem, or field. You will learn most of it as you go anyway.