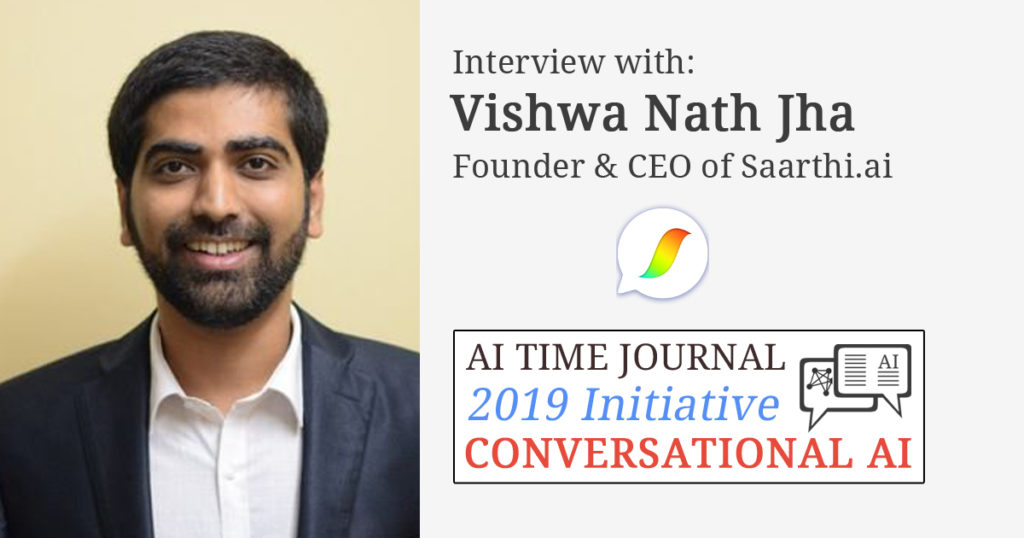
Vishwa Nath Jha is the Founder and CEO of Saarthi.ai, a company that develops a voice multilingual conversational AI platform that enables enterprises to automate customer engagement while reducing expenses, improving employees satisfaction, and, ultimately, customer experience.
In this interview, Mr. Jha shares practical insights on how enterprises can get started with conversational AI: from identifying which are the right challenges to face, how to gather the initial data, and ultimately, how the virtual assistant’s personality should be like to represent the company.
This interview has been featured in the Conversational AI Initiative 2019.
What is your background and how was Saarthi.ai started?
I’m Vishwa Nath Jha, founder & CEO of Saarthi.ai. I have done bachelor’s in information science & engineering and have been practising, teaching & consulting enterprises in AI, computer vision, speech & languages technologies for almost a decade now. I’ve trained thousands of professionals, students, faculties in data science, machine learning, NLP, etc. across the globe until now. Also, I advise the government of India in designing the policies and frameworks to expedite the research & entrepreneurship in Indian languages & speech technologies.
Saarthi was started with a purpose to offer a commerce enabled personal assistant for speakers of low resource Indian languages. In due course of our journey we’ve evolved into a B2B conversational ai platform focused towards working with customer obsessed enterprises having customer experience as top priority.
What is Saarthi.ai’s mission?
Saarthi’s mission is to empower enterprises with empathetic customer experience in indigenous languages.
Walk us through Saarthi.ai’s products, and what problems they solve.
Saarthi is a multilingual conversational ai developer platform built on top of a powerful state-of-the-art context-aware spoken language understanding engine. Saarthi’s multilingual conversational data annotator lets customer agents quickly annotate any historical or novel conversations for different downstream NLP tasks. Saarthi’s cognitive suite keeps track of signals such as emotion gradient, intonations, sarcasm, pitch, identity & functionality abuse, pre-emptive dialog breaks, etc. to generate near human responses.
Our conversational AI assistants eliminate long customer wait time, reduce average call durations and in necessary situations seamlessly transfer control to human agents who resolve customer queries through an agent friendly visual console. Human Agents can tag the novel conversations appropriately if deemed necessary for further improving the SLU & dialog models.
Saarthi’s speech based conversation analytics dashboard comes pre-loaded with 35+ key performance indicators which derive deep insights from the customer’s conversations & behaviour to improve the existing business processes. Our Call Quality Analytics dashboard gives feedbacks & suggestions to agents so that they can improve in the suggested areas and enhance the overall customer experience. Also, it provides interesting insights about customer’s behaviour.
As of now, we have developed end to end Conversational AI Assistants & Conversational IVRs for multiple clients across domains such as telecom, ecommerce, fintech, logistics, banking, etc. Few of the turnkey solutions include lead generation assistants, appointment booking assistant, order delivery & scheduling assistant, virtual HR, post-sale customer support, general FAQs, etc.
Today, enterprises have to reach customers to sell products and services on the channels where customers spend the most of their time. Consumers can be brought in the loop via conversational ai assistants and a sense of belongingness can be inculcated to increase customer retention.
What are the biggest challenges that you are currently facing at Saarthi.ai?
Let me begin with the current challenges faced in business. Despite the market being inundated with tonnes of bot companies globally, there is a huge lack of awareness among business owners in terms of expectation from the technology, associated cost and development time. This might be a result of poor previous experience with the technology partners. Conversational ai assistants get mature when fed with real conversations. The more conversations they handle, better they get in their language understanding and dialog handling capabilities. This customer education is one of the biggest challenges for me right now especially in Indian & South East Asian markets.
When it comes to technology, the biggest constraint is the lack of specialised conversational datasets for different NLP & speech tasks in languages other than English. Also, lack of enterprise grade Speech to Text & Text to Speech systems in low resource languages is also a major task for the research community. Another challenge that I feel worth mentioning here is the ever-growing cost of large scale computations involved in the development of conversational ai assistants. Right now, there is a strong trend of ongoing research in large language models due to their performance. However, this trend is not sustainable in the long term. Hence, achieving state-of-the-art performance with smaller model size (DistillBert, qBERT, etc.) is one of the important challenges that remains to be solved until today.
How can enterprises leverage conversational AI to make their customers happier and more satisfied?
Every business has customers and many customers reach out to businesses with their complaints, feedbacks or grievances. In the age of customer obsessed businesses, if customers are not attended with utmost care & attention, these customers are most likely to attrite and some may even speak negatively on social channels that’ll further cause damage to the brand reputation. Here, leveraging conversational ai to provide self-service to the customers during their entire lifecycle would be a great tool in customer success initiatives.
Enterprises need to understand the dynamics of information-driven decision-making behaviour of today’s consumers. Today, enterprises have to reach customers to sell products and services on the channels where customers spend most of their time. So, instant messaging channels like whatsapp, fb messenger, etc. are an integral part of customer success strategies. Be it marketing campaigns about a new product or a press release regarding a recent change in the company policies, consumers can be brought in the loop via conversational ai assistants and a sense of belongingness can be inculcated to increase customer retention.
In a world full of chatbots, an aspect of conversational ai assistants that becomes important is the agent personality as it carries a brand identity. Hence, enterprises need to carefully discuss and then decide an appropriate personality of the conversational ai assistant.
How can enterprises get started with their conversational ai strategy?
Conversational AI strategies depend on the data readiness of the enterprises. If enterprises have conversational data available from the historical live chat sessions or call transcriptions, things are lot simpler.
Data ready enterprises should begin with the identification of the busiest communication channels. Also, smart speakers should be discussed as a channel due to the growing adoption rate of the technology. Volumetric analysis of incoming queries per channel and average query resolution time for different queries should be assessed next. It’ll provide information such as top queries, their resolution time, no of different plots that need to be covered, important contexts, different intents, entities, etc. and help enterprises figure out the use cases & scope of work. This analysis can be done in-house with an experienced analytics team. However, I believe roping in a correct conversational ai partner for these analytics work is a better option because they can get their hands dirty with enterprises’ conversational data and identify a lot of useful conversational nuances due to their conversational ai expertise. Also, enterprises that are not data ready should leverage industry knowledge and consult conversational ai experts to discuss possible use cases and the implementation plans.
In a world full of chatbots, an aspect of conversational ai assistants that becomes important is the agent personality as it carries a brand identity. Hence, enterprises need to carefully discuss and then decide an appropriate personality of the conversational ai assistant. Also, enterprises should discuss on the key performance indicators of the assistants’ performance and also important analytical parameters to assess conversations & user behaviour history to enhance personalization.
With the advent of Smart Speakers, things have already changed a lot. These devices have opened up a new channel altogether and I strongly believe this channel will bring immense opportunities for enterprises to grow and reach their customers.
What are the biggest opportunities for enterprises to leverage Conversational AI?
Businesses in 21st century are all about providing unparallel personalised customer experiences. Today, providing personalised assistance and thereby building a strong promoter base on social channels are two major objectives for many enterprises. After all, these customer testimonials speak volumes to any prospective buyer/lead.
With the advent of Smart Speakers, things have already changed a lot. These devices have opened up a new channel altogether and I strongly believe this channel will bring immense opportunities for enterprises to grow and reach their customers.
Despite a huge acceleration in internet penetration globally, there are many markets with lower internet penetration. Here, conversational ai assistants instead of the decision tree-based Interactive Voice Response(IVR) systems can help enterprises reach new customers as well as engage with the loyal ones.
Which Conversational AI-related technology trend do you think will have the biggest impact in your industry in the coming years?
Almost every conversational AI agents we see today are goal-oriented dialog systems, i.e. they are designed to perform predefined business tasks. The major challenge with these agents is their inefficiency in handling out of domain queries. I believe, with mature Dialog Modelling algorithms overall conversational Ai experience for the end-users will improve immensely.
Also in a multilingual country like India, Code Switching & regional dialects of languages are a common phenomenon and hence, extending the capability of Spoken language Understanding to handle code mixed & dialect influenced voice input will also help in large scale adoption and open a largely untapped market for enterprises.
In the age of large language models ruling SOTA leaderboards, there is a huge need to focus on reducing the model size and inference time without losing on performance. Currently, huge costs for GPUs/TPUs are a barrier for conversational ai to penetrate the small and medium enterprise market.
What are the most interesting conversational AI use cases for business that you have seen emerging in 2019?
As per my experience, a few of the most fascinating Conversational AI Use cases for business this year are as follows:
- Call Centre Automation – General Enquiry, Appointments & Post Sales Support
- Patient Care – Drug Reminders & Data Collection for EMRs
- Supply Chain & Logistics – Order & Delivery Management
- Conversational Banking