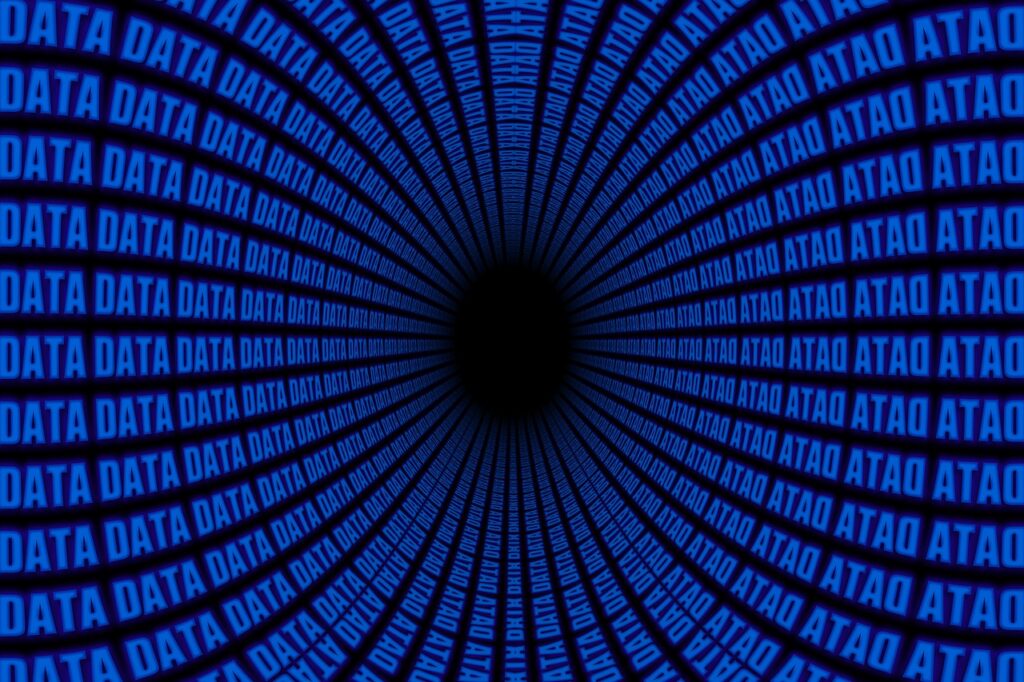
Once, data science was an untamed, emergent discipline without rules, restrictions, or repercussions. Organizations simply wanted accurate advanced analytics; those fortunate enough to procure scarce data scientists gave them boundless freedom in their sandboxes to manipulate data to achieve that end.
Nonetheless, the maturation of data science over the past 10 years has paralleled that of regulatory compliance for data privacy, data access, and data security. Today, data scientists, their sandboxes, and their tools are subject to the same regulatory scrutiny as other aspects of the enterprise, making it mandatory to implement data governance in this domain.
“With regulations and privacy, many organizations have changed,” admitted Privacera CEO Balaji Ganesan. “They no longer can have a very open culture around data. They understand that with regulations, data has some mandates that need to be met.”
Firms can readily meet these requirements for data science by following a three-step process of determining which data are sensitive, administering the proper controls around them, and instituting data lineage measures for ensuring those controls apply to downstream applications.
Those that fulfill these requirements will significantly reduce risk for devising advanced analytics via data science; those that don’t will proportionately expand their data governance risk.
Profiling Data
Once appropriate policies are created, the foundation for governing data science is based on discovering which data contain information warranting restricted access. Implicit to this step is the reality that certain information—such as a customer’s credit card number—is not only unnecessary, but also unnecessarily risky for creating sophisticated analytics solutions. “When you’re dealing with privacy data, you need to have visibility into what data is what: what is privacy data, and what is not,” Ganesan mentioned.
Intelligent data discovery approaches profile data to detail statistical information about them, such as which attributes relate to Personally Identifiable Information. This information, in addition to an examination of pertinent metadata, becomes the basis for classifying and cataloging data in accordance with established data governance protocols. The result is “really clear visibility of what is sensitive data and what is not through a profiling and discovery mechanism,” Ganesan said.
Obfuscation Controls
Once organizations determine which data are subject to data governance policies for data privacy, they must control how data scientists access them and their attributes. Effective controls for obfuscating data include issuing pseudonyms for some attributes and rendering others anonymous to preserve the security of sensitive information. Oftentimes, using both of these approaches is critical for layering the relevant data governance constructs to meet these demands. Specific obfuscation methods include:
- Masking: This technique involves data and their attributes stored as they originally appear. However, when a data scientist, for example, accesses that data, their sensitive attributes are obfuscated or masked to ensure data governance rules are met. In this case, the data are obfuscated “only for that person or that set of users,” Ganesan explained—who are data scientists, in this case.
- Tokenization: With tokenization, organizations create pseudonyms for aspects of data subject to governance mandates. Tokenization allows data scientists to “separate out the identifiers that can identify an individual person,” Ganesan commented. Identifiers include things like date of birth, social security numbers, email addresses, and other PII. Tokenization creates meaningless duplicates of this information that’s stored this way, regardless of who accesses it.
- Encryption: Encrypting data is another way to create pseudonyms for identifiers so data scientists don’t access them when using data in their work. These function as “alternate values to replace identifiers, which means they are unique,” Ganesan divulged. “I can replace a social with a unique number.”
Each of these approaches enables data scientists to access, query, and run analytics on data without violating data governance principles. Top solutions distribute these controls into source systems wherever data are.
Traceability and More
Data profiling lets organizations discover, classify, and catalog data according to data governance procedures for privacy and other concerns. Obfuscation controls limit access to such sensitive information while countenancing the use of that data for various aspects of data science. Data lineage or traceability provides a third layer of governance by delivering downstream measures for fortifying those controls—which is invaluable for data scientists deploying data for varying analytics purposes.
“If you copy something that’s already classified as sensitive, we can apply that to say this data is from existing data that’s already classified as sensitive, and we can classify the new data as sensitive,” Ganesan remarked. “And, all the controls associated with the sensitive label will move into the new data.” Thus, data scientists can readily replicate data into their sandboxes, tinker with them as need be, and even issue that data into production with cognitive computing models while adhering to data governance policies. This three-step approach is pivotal for ensuring regulatory compliance is met by data scientists while issuing the same stringent governance protocols in this realm as they are in any other.
Image Credits Featured Image: NeedPix