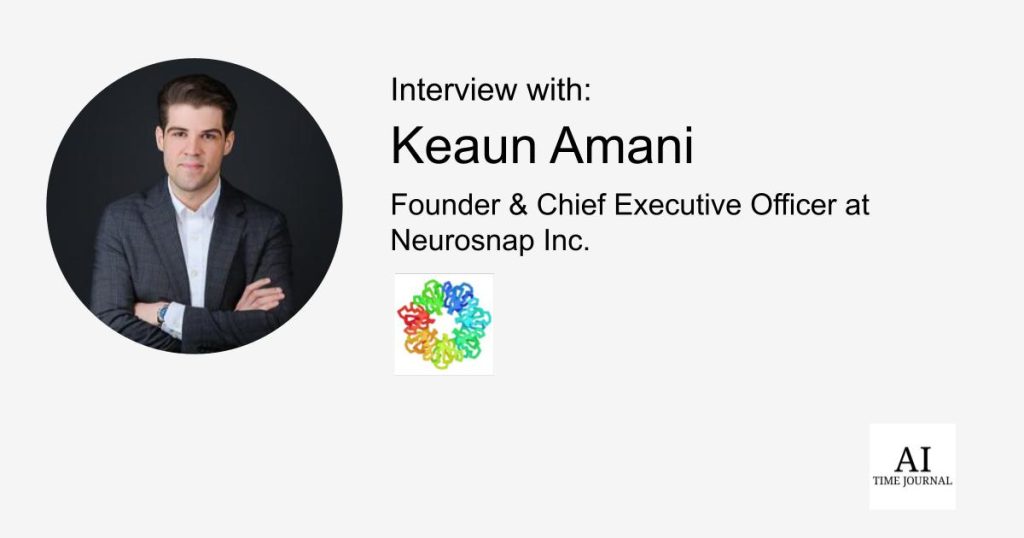
Keaun Amani, the Founder & CEO of Neurosnap Inc., stands at the forefront of integrating software engineering with molecular biology, tackling complex bioluminescent challenges through advanced AI. Amani’s unique interdisciplinary journey began during his university days, driven by a passion for both biology and computer science. His pivotal project on bioluminescent plants highlighted the inefficiencies in natural bioluminescence and the challenges in optimizing light-producing enzymes. Traditional methods like Deep Mutational Scanning (DMS) proved costly and time-consuming, spurring Amani to develop NeuroFold, an innovative enzyme design model. NeuroFold leverages a multimodal approach, combining various biological data sources, and significantly surpasses industry benchmarks in precision and efficiency. Under Amani’s leadership, Neurosnap has also launched a 2nd Generation Biology Suite with over 45 AI-based tools, enhancing research capabilities and democratizing access to bioinformatics. Amani’s vision for sustainable, eco-friendly innovations like bioluminescent plants and advanced AI tools continues to drive transformative progress in biotechnology.
Your background blends software engineering and molecular biology seamlessly. How did you first come to realize the potential for integrating these two fields, and what motivated you to pursue this interdisciplinary path?
I’ve always enjoyed biology and computer science, both fields are extremely unique in terms of their potential when it comes to leaving an impact. While growing up I spent a lot of time reading and trying to apply my knowledge in both fields but mostly separately. It was in University when I started working on my bioluminescent plant project where I really started seeing the potential for applying my knowledge in a joined way. For example, one of the biggest issues with natural bioluminescence is that the metabolic pathway necessary for the emission of light is somewhat inefficient which is why most bioluminescent organisms in nature are quite dim and difficult to see with the naked eye.
These metabolic reactions are catalyzed by special proteins known as enzymes and if you were to optimize the enzymes within the pathway responsible for producing light, you’d end up with greater light output and therefore a brighter plant. The only problem with this is that the optimizing and making enzymes faster is actually a really challenging problem and nobody’s really found a good way to do it. Most traditional approaches like Deep Mutational Scanning (DMS) basically involve making random mutations until you get something satisfactory.
The only problem with this is that for your average enzyme there are more possible mutations then there are atoms in the universe, and the vast majority of those mutations are deleterious meaning they either make the enzyme worse or completely non-functional. To make things worse the whole DMS process can cost hundreds of thousands of dollars, sometimes significantly more and the results can take years to manifest. This is was what led to the creation of our NeuroFold model which was designed to make precise mutations that lead to enzymes with specific and desired properties.
NeuroFold, your enzyme design model, has significantly outperformed industry benchmarks. Can you share the key innovations behind NeuroFold and its impact on molecular biology research?
The two key innovations behind NeuroFold are its multimodal approach to understanding the protein fitness landscape as well as leveraging a functional baseline. To expand on the first major innovation, multimodal models like DALL-E are essentially just models that receive more than two different types (aka modalities) as input. In the case of DALL-E, the model is able to receive both text and image data as inputs. While seemingly simple, this expanded context allows models like DALL-E to have a deeper understanding of our world as these machine learning models really only know about what they’ve been exposed to. The same concept can be applied to biological models as well.
Traditional approaches protein fitness prediction and enzyme optimization typically only focused on a single modality such as the sequence, evolutionary information, or structure. NeuroFold goes beyond and strategically leverages information from all three modalities in a concurrent way without “leaking” information from the other modalities. This gives NeuroFold a substantially greater understanding of the protein fitness landscape that no previous models were able to properly capture. Our other key innovation is to “bias” the model using an existing template. This one is a bit more complicated but bare with me. Most protein related models, especially protein language models (pLMs) tend to suffer from one of two major drawbacks, either they can’t really generalize to specific protein families or they can only generalize to a very select few protein families. This is because a very large portion of previous models were either trained on large datasets of proteins (e.g., sequences from UniRef) or trained on a dataset of proteins from a specific family. The advantage of the former is that the model can be trained once and then used by multiple researchers for many differing projects. The downside though is that the models tend to generalize poorly to certain types of proteins / families.
Alternatively training family specific models tends to perform better on the families they’re trained on but do worse on practically all other types of proteins. This also comes with the downside of having to train a new model for every different family you want to work with which isn’t ideal or accessible to most people. Some people also try to fine-tune already trained general purpose models with family specific data, a sort of middle ground between the two approaches. This unfortunately shares much of the same downsides as the 2nd option while also being increasingly more expensive and difficult to perform. NeuroFold doesn’t suffer from this critical flaw as the model is able to leverage a template protein that it then leverages as a reference to compare to. The model operates in a very unique way where constant comparisons to the template are critical to properly constraining the model into accurately understanding the intricacies of the input structure. This was what led to a 40-fold increase in performance when compared to Meta’s ESM-1v model.
Neurosnap’s new 2nd Generation Biology Suite includes over 45 innovative AI-based tools. How do these tools specifically enhance the research capabilities of scientists, and what unique advantages do they offer over existing solutions?
Our 2nd generation software suite features over 46 AI tools and models designed to accelerate research across a broad number of tasks in molecular biology. Some of the most prominent changes consist of improvements and optimizations to tools like AlphaFold2, as well as the addition of new tools for drug and protein design.
Your work in synthetic biology includes engineering bioluminescent plants. What inspired this project, and how do you envision such innovations contributing to sustainable and eco-friendly technologies?
My inspiration for creating bioluminescent plants actually stemmed from a failed kickstarter that happened several years prior. Bioluminescence in general is a truly remarkable and not to mention beautiful phenomenon to witness. Despite this, there are surprisingly no naturally occurring plants that possess this trait. But I figured if mushrooms, algae, insects, and even fish could all pull off their own distinct versions of bioluminescence, then it must be possible for plants as well.
Long story short, I think a glow in the dark willow tree would not only be extremely cool, but also shape the way for unique plant based decor and eco-friendly lighting solutions. Afterall, the bioluminescent plants we created not only produce light visible to the naked eye but also purify the air by removing carbon dioxide and producing fresh oxygen.
Neurosnap aims to eliminate the need for researchers to do computer coding. Can you discuss how this approach democratizes access to advanced bioinformatics tools and the potential it has to accelerate scientific discoveries?
Tools like AlphaFold2 are in my opinion, among the most revolutionary models in this space as they not only drastically improve scientists’ ability to quickly reason about a proteins structure but it also invigorated interest in the computational biology space leading to a number of exciting models and tools coming out as well. Protein folding, traditionally, had been a vital component to a lot of research in molecular biology. It’s an extremely common process and it’s also extremely time consuming, expensive, and laborious process. It could cost thousands of dollars, requires very specialized personal and equipment, could take months to perform, and you’re not even guaranteed to get any worthwhile results out of it.
For comparison, using the Neurosnap AlphaFold2 implementation, researchers can perform virtual protein folding in a span of minutes to hours with a pretty high degree of accuracy at effectively no cost. Best of all, we add additional confidence metrics on top of AF2’s own metrics, allowing scientists to reliably assess whether or not the production is accurate. Best of all, this can be done in parallel with traditional methods allowing for even more reliable results and insights.
As someone who transitions effortlessly between academia and industry, what are the main differences you perceive in the approach to innovation and problem-solving in these two environments?
I would say the biggest difference between academia and industry is that in industry the biggest priority is to create a functional and safe product that you can then get a return on. Whereas in academia it’s more theoretical and the main driving factors for academics is to create novel and exciting research that will ideally yield positive attention on their research as well as yield more citations. This difference means that in general academics tend to be more open with their research as it not only benefits the scientific community as a whole but also their reputation within it. Industry on the other hand tends to be a bit more private with their research as companies aren’t publicly funded institutions and hence need to protect their bottomline. In terms of research methods employed, both are quite similar and the bigger differences tend to come from the lab’s research budget.
The latest tools in Neurosnap’s platform include improvements in protein folding prediction accuracy and efficiency. What are the most significant advancements in these tools, and how do they influence the research process?
For protein folding specifically, we have added additional metrics to models like AlphaFold2, RoseTTAFold2, ESM-Fold in the form of the uncertainty metric as well as the pDockQ score. The Uncertainty metric is a proprietary metric we developed at Neurosnap for AlphaFold2 thathelps sample the model’s uncertainty or lack of confidence within a predicted structure. This can be really helpful to researchers as sometimes you might get a plausible looking structure that is incorrect and it is critical to know exactly when we should be trusting these structures. The pDockQ score is an optional metric we calculate for assessing the quality of multimers.
Multimers are essentially just complexes consisting of at least 2 or more proteins and we found that more often than not, people don’t just want to predict a single protein structure but also how that protein folds in the presence of other proteins.
For that reason we decided to add the pDockQ score which is a very cool metric developed by the authors of the nature paper Improved prediction of protein-protein interactions using AlphaFold2. Lastly AlphaFold2, can be quite sensitive to the input multiple sequence alignments (MSA) it receives as input. By building upon research from the ColabFold team as well as the latest CASP15 results, we have found ways to improve MSA quality without significantly impacting prediction time.
Looking forward, what are some of the most exciting developments or projects at Neurosnap that you believe will redefine the future of molecular biology and drug discovery?
Our next biggest initiatives are going to be expanding upon the success of our recent R&D projects like NeuroFold as well as to create new tools for improved antibody design. We strongly believe that antibodies are going to play an enormous part of the therapeutics landscape and we’re willing to back that belief with our research.
Your journey as a polymath and innovator is truly inspiring. What personal philosophies or principles guide you in your work, and how do you maintain a balance between your diverse interests and professional commitments?
Lucky for me, my interests are fully aligned with my professional commitments. I truly do enjoy the work we do at Neurosnap as it gives me the opportunity to not only research areas at the intersections of biology, computer science, and data science, but also the chance to help my fellow researchers in those areas as well. Every day at work is unique and provides its own interesting challenges, which is something I not only enjoy but also pride my colleagues on.
As for my personal philosophies. I believe that hard work, consistency, and determination are key to success. I’m also a big believer in good luck and I would highly recommend those with grandiose aspirations to try everything they can to maximize these serendipitous events. Lastly, I believe that surrounding oneself with quality individuals is also critical to success, not just commercially, but also academically / in research. I am very grateful to my colleagues, both new and old, and that their feedback and guidance has been indispensable.
AI is rapidly transforming various sectors. In your opinion, what are the most promising applications of AI in biotechnology, and how is Neurosnap leveraging these opportunities?
Given current trends in biotech, I strongly believe that the protein design market is going to rapidly grow over the next several years. Proteins are remarkable and incomprehensibly diverse in terms of functionality and use cases and we’ve seen a significant increase in protein design related efforts globally over the last several decades. Not to mention, platforms like Neurosnap drastically lower the barrier of entry for protein design related tasks make it far cheaper, faster, and more accessible to perform tasks like enzyme, peptide, or even antibody design using our tools and models.
Additionally, antibody based therapeutics are among some of the best in industry. The problem though is getting them to work in a safe and effective way is extremely challenging. This is also why we’ve also shifted many of our new tools to be as helpful as possible for antibody design.
Given the exponential growth of technology, where do you see the intersection of AI and biotech heading in the next decade, and what role do you envision Neurosnap playing in that future?
Right now we’re truly fortunate as we’re almost living through a computational biology renaissance or even golden age. Every few months we see new models push the boundaries of what we thought was possible in bioinformatics and we’re extremely excited to see these AI based tools shape the biotech and pharmaceutical industries. As for Neurosnap, we’re going to continue doing what we do best and focus on keeping our platform great and user friendly, while also strategically investing in developing new tools and models that will provide value to our customers.