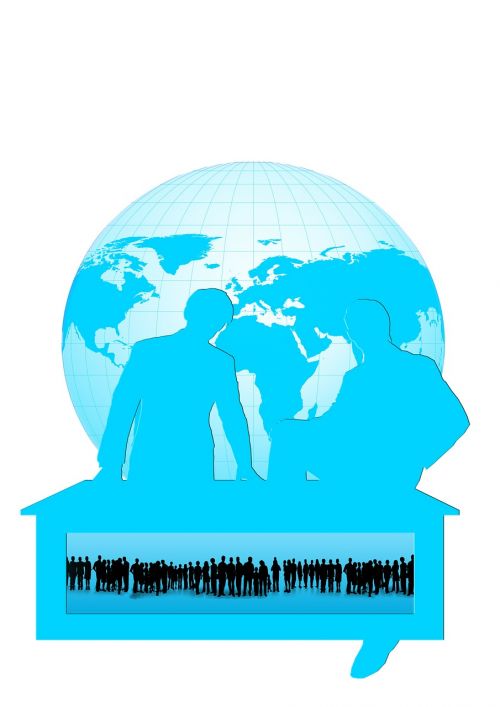
Data security and access governance has become one of the cardinal points of commonality for all organizations. The need to ascertain where one’s sensitive data is, administer the proper controls to protect it, and demonstrate doing so with timely auditing is pivotal to survival in today’s hyper-regulatory compliant business conditions.
But just as data governance itself is transitioning into a discipline almost singularly focused on access controls, security, and data privacy, its capabilities to fulfill these objectives are evolving.
Traditionally, this area of data management relied on historic or low latent capabilities for securing data access. Tomorrow, accomplished governance platforms will increasingly involve predictive and prescriptive functionality to enable users to govern their data for the future.
According to Privacera CEO Balaji Ganesan, “What customers really want is: hey, tell me today who’s got access to what data. Tomorrow, flag any potential compliance violations. Flag any security violations, and be proactive about it.”
That’s just what elite governance solutions are doing with an artful combination of machine learning, cognitive computing, and data provenance. Combining these and other elements enables organizations to swiftly understand next best actions for meeting their governance requisites—and implement them to mitigate any forthcoming risk.
“Now that we’ve got all the data, we can not only give…reporting and visibility, we can monitor that data and continuously look for some things that could potentially lead [users] into some challenges, whether that’s a compliance or security violation,” Ganesan remarked.
Supervised and Unsupervised Learning
One of the first procedures for facilitating predictive and prescriptive data security governance involves centralizing access (and controls) to distributed data sources. Most modern governance solutions have measures for determining sensitive data and providing a central means of accessing it with attendant monitoring capabilities for data stewards. The next step entails automatically generating suggestions for compliance via machine learning to preserve data privacy, regulatory compliance, and data governance. “This is a huge value add for the enterprise, because it’s not one of their core competencies and it’s hard for them to do manually,” Ganesan observed.
Systems with supervised and unsupervised learning can establish a baseline for user behavior and provide anomaly detection that alerts governance personnel of possible policy violations. “Because we have lots of data, we can make inferences and provide that value in a model, which is trained on that, to our customer,” Ganesan commented. Once those models are incorporated into customer settings, they naturally adapt themselves to the behavior of specific users or use cases. For example, if analysts typically access datasets three times a day, and then the number of times skyrockets, machine learning algorithms can flag such behavior to send alerts to the proper personnel. “It gives them a point of view to take a look, because there’s so much going on they can’t just access everything,” Ganesan said.
Data Provenance
Platforms that involve aspects of machine learning with data lineage can notify users of other developments germane to data governance personnel. One such subtlety that may easily become a point of concern is the amassing of access privileges for particular users, sources, or datasets. “If an analyst has not accessed some data for six months or three months, we can say, do they still need access to that?” Ganesan mentioned. “If you ask them they’ll still say yes, but now you have data to prove that you can go and compress that part of it.”
Data provenance is particularly useful for detecting compliance violations—which organizations can determine and remediate before regulators become aware of them. For instance, some organizations have certain sensitive data in restricted zones for regulatory adherance. Were a data scientist to potentially move such data into his or her sandbox to build features for a machine learning model, governance systems scrutinizing data lineage could identify this action and surface alerts about. “We would track, from a lineage point of view in those cases, to say this data has moved from a restricted zone to a sort of a public zone,” Ganesan denoted. “It’s not bad, but it’s a compliance violation. It’s against the rules and you need to have a conversation with the data scientist.”
The Might of Automation
Technically, data lineage is part of the historic-facing capabilities of data security and access governance solutions. However, applying it to the use case Ganesan articulated above illustrates how it can still negate what would otherwise be a future compliance violation, penalty, or lawsuit. Pairing this functionality with machine learning to evaluate user behavior and identify anomalies enables organizations to create next best actions to successfully govern their data for the future.
Featured Image: NeedPix