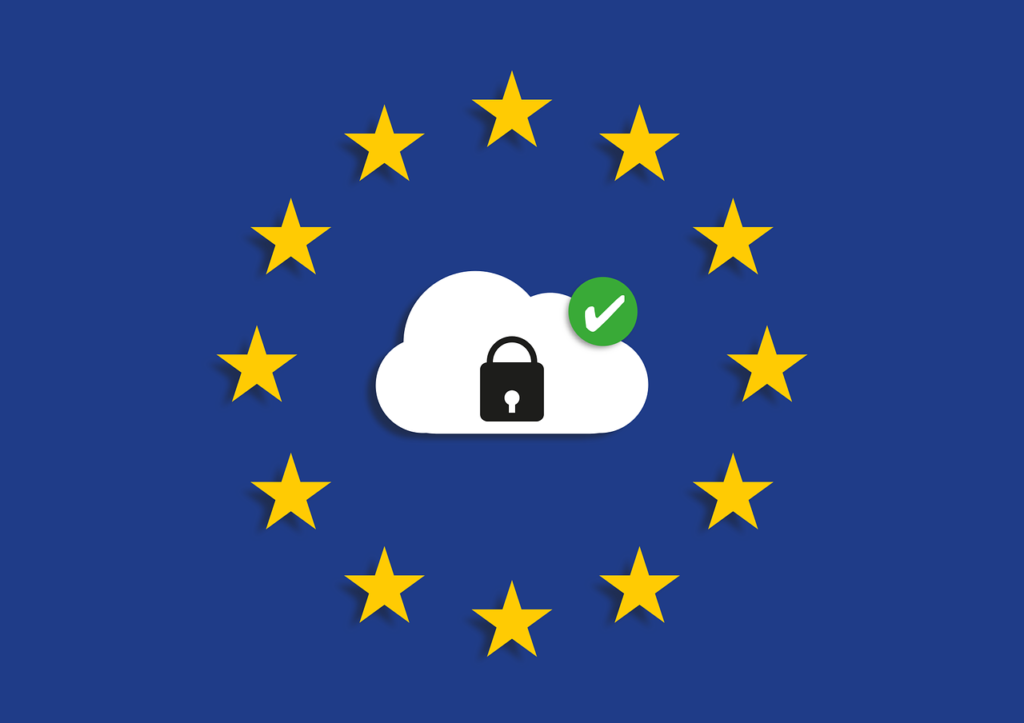
In the hierarchy of data governance personnel, data stewards have traditionally had the least amount of influence in devising the policies that dictate how organizations govern their data. The Chief Data Officer (CDO) typically has the most sway, followed by that of data governance councils.
Even the input of business users stipulating various data requirements is more valued than that of stewards, who are mainly tasked with ensuring policies are properly implemented.
However, several factors have contributed to a new—and higher—valuation of data stewardship, specifically in relation to tailoring governance policies throughout the enterprise. These include:
- Statistical Artificial Intelligence: Machine learning and other forms of statistical AI require copious quantities of data to train and refresh models. This reality expands the amount of data organizations are accountable for, which produces a similar effect on their need for effectual governance.
- The Decentralization of the Data Landscape: If AI increases the quantity of data organizations must govern, the distribution of the data landscape to various clouds, edge applications, on-premise deployments, and remote work options broadens the surface area of what data governance encompasses.
- Regulatory Adherence: The number, severity, and penalties for regulations are escalating in almost every area of data management, particularly in terms of data privacy.
These developments are rapidly transforming how organizations govern their data and the roles data stewards play in their doing so. These factors are directly responsible for what Syed Mahmood, Privacera Product Marketing, referred to as the delegated model of data governance in which “IT is saying we understand tools, so we will give [the business] a platform that you will use to govern your data. But, you understand your data, so your data stewards within each line of business can configure the policies according to your own needs.”
Delegated Data Governance
The delegated data governance approach relies on data stewards for three reasons. Stewards (1) understand the underlying data business users require, (2) know how it relates to their use cases, and (3) are cognizant of which governance policies relate to that data and its use cases.
Therefore, they can tailor those policies and provide the requisite access to specific business users to greatly expedite the time it takes to use data—especially when compared to centralized governance models in which IT teams (that don’t understand the data) are positioned between the business and data access. Although there are plentiful benefits to this approach, specific ones related to data science include:
- Celerity: This approach intrinsically yields “faster access to data,” Mahmood disclosed. “Data is the raw material and organizations take that data and convert it into analysis, data models, predictive models, and machine learning models.”
- Productivity: The reduction in time-to-data optimizes investments in data scientists. Mahmood characterized these professionals as “very expensive resources, data scientists and business analysts, and the longer they’re sitting around waiting for the data to arrive, the longer they’re being unproductive.”
- Trust: With the delegated model, the data lineage that’s critical for understanding outputs of so-called ‘white box’ machine learning models begins with data stewards effectively curating policies for data scientists as data consumers, because the former “understand data, what is the context, and what is the trusted source of data,” Mahmood noted.
Governed Data Assets
By delegating greater responsibility to data stewards for determining how centralized governance policies are actually carried out in individual business units, organizations achieve a couple objectives. Firstly, these succeed in distributing the focal point of centralized policies to individual business units—which is aligned with the decentralization of the data landscape and the heterogeneous tools and resources with which those units manipulate data. Secondly, they improve the overall quality, worth, and trust in data as established enterprise assets, much like any other, in which governance is ingrained.
Therefore, end users “get back from the data owner this data asset and it is with the assumption that all the access control policies have already been applied to it and I can start using it,” Mahmood mentioned. “So, all the complexities of access control policies, permissions, and privileges have been hidden from me.” Best of all, that data can stem from myriad sources distributed throughout the data ecosystem. Users can ensure data assets have already been vetted for common governance concerns. “Rather than cloud based services, these are data assets that I need as an analyst or a data scientist to do my job,” Mahmood specified.
Contemporary Data Stewardship
Although users are oftentimes accessing cloud services to obtain the data they need, with the delegated model the particulars of those concerns are handled by data stewards. These professionals tailor centralized policies for individual lines of business or data scientists, reinforce them, accelerate access to data, and facilitate trusted data assets across sources and settings. Stewards are now at the forefront of properly configuring data governance for the challenges of the modernized, highly dispersed data ecosystem.
Featured Image: NeedPix