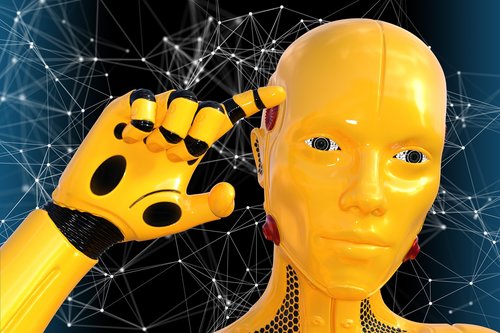
As a concept, intelligence has myriad connotations, denotations, and contexts. When applied to the notion of Artificial Intelligence, however, the most prominent of these considerations is the capability for machines or ‘smart’ systems to a) learn and to b) reason.
Although these tenets may sound similar to one another, they’re actually distinct and form the basis of true AI, which combines them for astounding results in applications of natural language technologies, conversational AI, and cognitive search.
Most people are fairly familiar with AI’s learning capacity as exemplified by machine learning or deep neural networks. However, it’s capacity for reasoning—to comprehend the semantics of terminology or enterprise knowledge, represent it, and make shrewd inferences about it to create additional knowledge—is equally vital in the aforementioned use cases.
“People don’t consider Google’s knowledge graph AI at all like, ‘that’s not AI’,” observed Kyndi CEO Ryan Welsh. “Like AI must very specifically become a learning system as opposed to a reasoning system which is funny, because those learning systems have no capacity for reasoning at all. But that [Artificial] Intelligence, part of it is reasoning.”
Reasoning is a vital aspect of AI for rapidly profiting from enterprise knowledge and applying it to real world questions and answers via natural language.
Symbolic AI
Neuro Symbolic AI is the term for synthesizing AI’s reasoning capabilities with its machine learning ones for total or complete AI. It’s predicated on AI’s knowledge base (which frequently relies on knowledge graphs, terminology systems, and extensive data models called ontologies encompassing terms and their meaning) in which it understands critical business concepts. This semantic comprehension is pivotal for understanding what various words mean in terms of their enterprise value to business users, which then become the basis for intelligently reasoning about them.
“Chompsky would say that language has hierarchy; it doesn’t just have relatedness,” Welsh specified. “So, take an example of like ‘laptop’ and ‘computer’. A laptop is a type of computer. A computer isn’t a type of laptop. A pure vector based approach would just say hey, these two things are similar.”
In this respect, the knowledge underpinning reasoning systems provides more granular, nuanced understanding of terms and their meaning to the enterprise—which delivers more advantageous search results and natural language query results than do systems that rely disproportionately on the machine learning vectors Welsh referenced. The reasoning facilitated by knowledge graphs and their atomic understanding of enterprise terms for any application of natural language technologies are critical for this variety of AI—particularly when it’s paired with its learning dimension.
Statistical AI
The ability to fuse and cull from AI’s reasoning and learning foundations at will consistently delivers the best business outcomes for search or conversational queries in natural language. According to Welsh, utilizing an assortment of AI techniques—as opposed to just one—is essential for optimizing these applications, reducing both the time and cost it takes to employ them.
Credible Neural Symbolic AI solutions are “so well versed in so many different techniques,” Welsh mentioned. “I think it was Minsky out of MIT where, when asked about what makes the brain work, he was like the trick is that there is no trick. There’s a bunch of different types of learning and reasoning systems and all these different agents, he called them, that come together to solve the task at hand.” Consequently, top options in this space avail themselves of machine learning (via supervised and unsupervised learning techniques) to assemble the requisite knowledge necessary to understand a corpus of documents organizations want to search through, for example.
These learning capabilities can swiftly perform such functions at enterprise scale to assemble the knowledge for AI systems to understand and acutely reason about to answer queries in a brief amount of time—particularly when compared to more traditional methods of constructing exhaustive taxonomies beforehand, or using purely supervised learning approaches requiring extensive, time consuming data labeling. “It’s a usable system out of the box,” Welsh commented about the Neuro Symbolic AI method. “You can tune it to get even better, but it’s ready to go out the box.”
Enterprise Ready
Ultimately, the capability to immediately begin asking, answering, and parsing through text in natural language is perhaps the supreme advantage of uniting AI’s reasoning and learning dimensions in a single solution. Aside from the obvious business value implicit to this reality, the greater gain is maximizing the enterprise worth of AI—which doesn’t just hinge on machine learning applications, but on using them to reinforce AI’s knowledge base (and vice versa).
“For the demerits of each approach—the demerits of machine learning and connectionist approaches, and the demerits of symbolic approaches—the merits of each approach align with the demerits of each approach,” Welsh noted. “So it’s kind of like how do you fuse these two together to get a system that’s more powerful than the individual approach? And, that’s ultimately what we did here at Kyndi.”
Featured Image: NeedPix