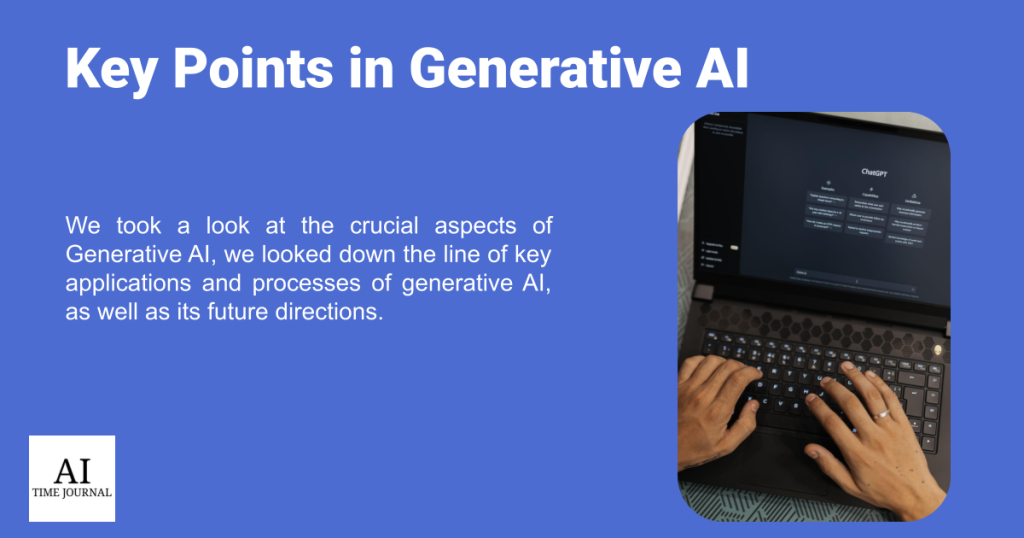
Generative AI is a class of artificial intelligence techniques that contain creating or generating new data, content, or outputs that are similar to already existing data. These techniques are mainly used in fields such as computer vision, art generation, natural language processing, and machine learning. The main goal generative AI serves is to enable machines to produce outputs to exhibit characteristics akin to human-created content. The generator creates new data instances, while the discriminator evaluates them for authenticity.
Generative AI serves a crucial role in modern technology due to its ability to provide new and meaningful content. As the co-founder and CEO of Space X and Tesla Elon Musk says “Generative AI is the most powerful tool for creativity that has ever been created. It has the potential to unleash a new era of human innovation.”
Table of Contents
- Generative Adversarial Networks (GANs)
- Text Generation and Natural Language Processing (NLP)
- Creative Applications
- Medical and Scientific Innovations
- Privacy and Ethical Considerations
- Deepfake Technology
- Cross-Domain Translation and Style Transfer
- Real-World Applications
- Future Directions and Challenges
- Conclusion
Generative Adversarial Networks (GANs)
A GAN consists of two neural networks, the generator, and the discriminator. These two networks are trained simultaneously. The generator creates new data instances, while the discriminator evaluates them for authenticity. As the training moves forward the generators aim to produce data that is increasingly difficult for the discriminator to distinguish from real data. GANS are being used for style transfer, image synthesis, and even generating realistic human features.
The Generator-Discriminator Architecture is a fundamental notion in generative AI and a key component to GANs as talked about in the paragraph above when they are pitted against each other in a competitive process they create highly realistic data.
Generative Adversarial Networks (GANs) have revolutionized the field of image and content generation. They excel at creating realistic and high-quality data, making them particularly well-suited for tasks that involve generating images, videos, and other forms of content.
Text Generation and Natural Language Processing (NLP)
Text generation is a pivotal application within the space of Natural Language Processing (NLP). AI Models for Text Generation are designed to produce coherent and contextually relevant human-like text based on various inputs. Many of the AI models out there have been developed for text generation, each one with its own characteristics and approach. Some notable models of text generation include Recurrent Neural Networks (RNNs) which are a class of neural networks that can process sequences of data, making them suitable for text generation tasks, Long Short Term Memory (LTSM) which Is an advanced type of RNN that addresses the vanishing gradient problem by using gating mechanisms and Variational Autoencoders (VAEs) which generate models that can learn latent representations of text data.
OpenAI’s GPT Series has been a groundbreaking set of language models that have substantially advanced the field of natural language processing and text generation. The Generative Pre-trained Transformer models have been assembled upon the transformer architecture and have demonstrated incredible capabilities in creating coherent and contextually relevant text. Starting from the original GPT-1 model in 2018 up until now we have GPT-3.5 which built upon the strength of its predecessors.
Language Translation and Sentiment Analysis are two essential applications of the NLP that involve the process of understanding and processing the human language.
- Language translation is a process of converting text or even speech from one language into another at the same time preserving the meaning and context. NLP models assist in automating this process.
- Sentiment analysis, also known as opinion mining, is the process of determining the sentiment or emotional tone expressed in text. It involves classifying text as positive, negative, or neutral. It has various applications, including social media monitoring, brand reputation analysis, market research, and more.
Creative Applications
Generative AI has had a monumental impact on the fields of art and music, enabling innovative and creative applications that will push the limits of human creativity. Generative AI has the ability to produce unique and original artworks with a wide range of variety which include abstract composition pieces all the way to intricate designs.
Musical Generative AI is another incredible application AI has to offer as it can compose original musical pieces in various genres and styles AI can also generate MIDI sequences that can be used as musical building blocks to help musicians and producers in their creative process. Mimicking styles is also another unbelievable feature of generative AI, AI can mimic the musical styles of different composers, artists, or eras, allowing musicians to experiment with a wide range of musical influences. Popular American Musician Post Malone talks about mimicking style on the Joe Rogan Experience Podcast and said:
“You can still tell if it’s an AI song right now, but the way that it’s moving like I don’t know. My buddy Lou Lewis Bell produces a bunch of my stuff, and we write together he’s the most talented smart dude ever, but he taught me about epochs and how many epochs a better AI template is, and pretty soon dude it’s gonna be really really good, it’s just going so fast”. Post Malone
Generative AI has also found innovative applications in the field of fashion design and textile creation. It has the ability to offer designers new tools and methods to explore novel patterns, designs, and materials.
Generative AI has begun to play a significant role in game design and the creation of virtual worlds. This technology enhances various aspects of gameplay, visual aesthetics, and player experiences. An example of AI’s important role in game design can be seen in terrain and world generation. AI can create expansive and diverse virtual landscapes, regions, and worlds, this is most useful in open-world games where every inch, every pixel on the screen is impractical.
Medical and Scientific Innovations
The use of Generative AI has greatly impacted the field of drug discovery and molecular design. It was able to do this by accelerating the process of identifying likely drug candidates and novel molecules with fitting components.
Molecule generation works by Generative AI models, such as Variational Autoencoders (VAEs) and Generative Adversarial Networks (GANs), generating new molecular structures based on existing chemical knowledge. These models learn from large databases of established molecules and generate novel compounds with desired properties.
Another amazing medical innovation Generative AI brings to the table is enhancing medical imaging and diagnosis by enhancing image quality, helping in image analysis, and assisting medical professionals in making accurate diagnoses.
Some keys to how Generative AI is enhancing medical imaging and diagnosis include:
Generative AI has also taken huge steps in the field of material science, by revolutionizing the way new materials are being discovered, designed, and enhanced. Generative AI has the amazing ability to navigate complex structural and chemical spaces which substation ally accelerates the materials discovery process. Leading to the development of advanced materials with applications across vast industries, ranging from electronics, energy, healthcare, and manufacturing.
Privacy and Ethical Considerations
While Generative AI tech is revolutionary there are some potential privacy risks and raise important privacy concerns due to its ability to create human-like content. One of these privacy issues is impersonation and phishing, advanced text and voice generation promises could enable more sophisticated phishing attacks, where attackers impersonate known individuals, and create emails or messages that seem genuine but are actually malicious. Social engineering attacks also could use generative AI to create realistic messages, images, or videos to manipulate individuals into taking actions they wouldn’t otherwise.
A crucial aspect of dealing with generative AI is Ensuring Responsible Data Handling to lessen ethical concerns, privacy risks, and potential misuse of AI-generated content. Two key practices that must be considered are Data Privacy and security, as well as ethical data collection and usage. The best way to are listed in the bullets below:
- Anonymize Data
- Data Encryption
- Access Control
- Ethical Data Collection and Usage
- Ethical Guidelines
- Informed Consent
- Bias Mitigation
Mitigating bias and unintended consequences in generative AI is another important step to ensure fair, ethical, and responsible use of AI-generated content. This can be done by providing Diverse and Representative Training Data, Ethical Guidelines and Governance, and Responsible Deployment.
Deepfake Technology
Generative AI also has great potential with deepfake technology. Deepfakes refer to manipulated digital content, often videos, images, or audio, that are generated using advanced artificial intelligence techniques, particularly deep learning algorithms. These techniques involve training deep neural networks on large datasets of real content and then using these networks to generate highly convincing, yet fake, content that appears authentic and realistic. The term “deepfake” is a combination of “deep learning” and “fake.”
Deep fake technology is truly amazing but there are always two sides to the coin, here are the positives and negatives Positive and Negative Use Cases
Positives:
- Entertainment and Film Industry
- Voice Acting and Dubbing
- Digital Art and Creativity
- Education and Historical Recreation
- Disability Support
Negatives:
- Misinformation and Fake News
- Fraud and Identity Theft
- Reputation Damage
- Manipulation of Evidence
- Privacy Violations
- Disinformation Campaigns
- Cybersecurity Threats
- Political Manipulation
- Media Authenticity Concerns
Here is how we can try to combat deep-faking problems with Misinformation and Manipulation. It will take a multifaceted approach that involves technology, education, policy, and public awareness. Here are three strategies that are already being deployed to combat this issue: blockchain technology, advanced Detection Technologies, and Media Verification and Authentication.
Cross-Domain Translation and Style Transfer
Generative AI’s ability to translate content between different domains is a powerful and innovative application that can help bridge the gap between various types of data, enabling new possibilities for creativity, research, and problem-solving.
Applying Artistic Styles to Different Media Artists can apply different artistic styles using generative AI involves leveraging style transfer techniques to transform the characteristics of one type of content into the style of another. We will be able to choose the content you want to transform (text, music, images) and then choose the artistic style you want to apply which can be a famous painting, a specific writing style, or a musical genre)
Generative AI opens up a world of creative possibilities across various domains, empowering creators to explore new avenues of expression and innovation. Generative AI’s ability to push creative boundaries comes with the responsibility to ensure ethical, diverse, and unbiased outcomes. Collaboration between AI developers, creatives, ethicists, and end-users is essential to harness the full potential of generative AI while maintaining ethical considerations and quality standards.
Real-World Applications
Generative AI has impactful applications in the development and enhancement of autonomous vehicles and simulations. Generative AI is contributing to safer, more efficient transportation systems and improved training environments. AI’s capacity to develop realistic scenarios that accelerate the development of autonomous vehicles and will enable invaluable tools for training, research, and decision-making worldwide.
Virtual assistants and human-machine interaction are domains that are greatly impacted by generative AI. Generative AI enhances communication, personalization, and convenience in various applications. We took a look at some of the Generative AI influences on virtual assistance.
- Conversational AI- Generative AI powers natural language processing models that enable virtual assistants to engage in human-like conversations, providing users with information, assistance, and entertainment.
- Personalization- Virtual assistants use generative AI to learn from user interactions, tailoring responses and recommendations based on individual preferences and historical data.
- Task Automation- Generative AI can automate tasks based on voice commands, such as setting reminders, sending messages, or controlling smart home devices.
Personalization in marketing and recommendations is a significant application of generative AI that aims to tailor content and suggestions to individual preferences, enhancing customer engagement and driving sales. Dynamic ads are a very interesting way generative AI influences marketing personalization. AI-generated content enables dynamic ad campaigns that change in real time based on user behavior, improving relevancy and click-through rates. Another incredible feature Generative AI offers is predictive analysis. This tool will help marketers predict customer behavior and preferences, helping marketers create campaigns that align with customers’ likely interests.
Future Directions and Challenges
Generative AI is continuing to advance propelling the field forward, leading to increasingly sophisticated models and applications across various domains. The model scale and complexity of generative AI are continuing to grow enabling more accurate and detailed outputs in tasks like image synthesis, text generation, and content translation.
There are many other future applications of generative AI, we encourage you to check out this article from Forbes on the future of generative AI.
As amazing as generative AI is it still presents many ethical challenges considering the powerful impact this tech has on society. Here are some of the regulations that can combat the potential challenges Generative AI brings.
Challenge – Misinformation and Deepfakes
Regulation – Some jurisdictions are exploring regulations to address deepfakes, including labeling requirements for synthetic content and penalties for malicious use.
Challenge – Privacy Concerns
Regulation – Data protection laws, such as GDPR, may extend to AI-generated content, requiring consent for its use and protecting individuals’ privacy.
Challenge – Technological unemployment
Regulation – Policymakers may need to explore strategies to mitigate job losses through upskilling, reskilling, and workforce transition programs.
Conclusion
Collaboration between AI and humans, often referred to as “human-AI collaboration,” involves leveraging the strengths of both entities to achieve more effective, efficient, and innovative outcomes. This collaboration can occur across various domains and industries. Effective collaboration involves clear communication, understanding each entity’s strengths and limitations, and integrating AI as a tool that enhances human capabilities. Ethical considerations, data privacy, and accountability are also crucial in maintaining a balanced and responsible collaboration between AI and humans.