Industry Background
Today, companies are using AI in every aspect of their businesses. The adoption of AI has been very influential especially in the finance and healthcare sectors, where the impact of implementing AI solutions is very significant. In the financial services, AI is playing a very important role to optimize processes ranging from credit decisions to quantitative trading to financial risk management. In healthcare, we are seeing a spike in adoption of conversational bots, automated diagnoses and predicting diseases.
This increase in adoption is also causing a cultural shift in our relationship with AI. As AI systems become more ubiquitous, they are coming under a spotlight of strict scrutiny. Governments are calling for regulating these AI models, discussing their ethical impact on our society and giving users rights to demand an explanation for an automated decision. This shift highlights the question of trust, transparency and explainability of these AI systems, that so far have been black boxes – we don’t know how they come up with an answer.
As artificial intelligence (AI) systems gain industry-wide adoption and the government calls for regulations, the need to explain and build trust in automated decisions by these AI systems is more crucial than ever.
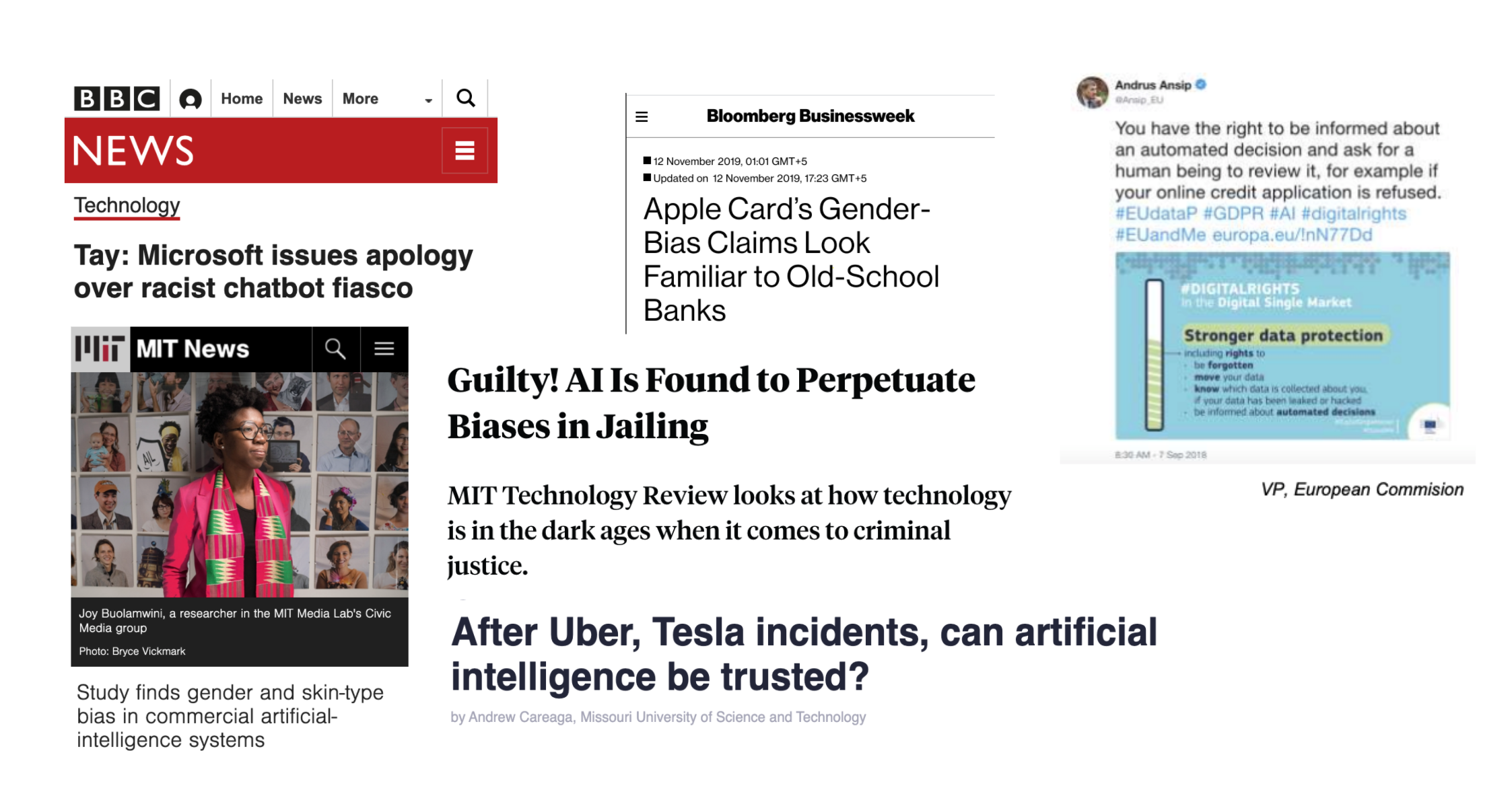
Enter Explainable AI
Explainable AI (xAI) or interpretable AI addresses the major issues that stop us from fully trusting AI decisions and through its practical applications, can help us ensure better outcomes.
With practical explainable AI, we are able to explain the “why” and “how” behind AI decision making in a palatable and easy to understand manner. We have to remember that explainability is extremely crucial in systems that are responsible for carrying out mission-critical tasks. For example, in healthcare, if scientists are relying on AI models to help them figure out whether the patient will have cancer or not, they need to be 100% sure of their diagnoses otherwise this can result in death, a lot of lawsuits and a lot of damage done in trust. Nature is this problem is so intense that explainability sits at the core of this problem: the data scientists and the human operators, in this case, the doctors need to understand how the machine learning system is behaving and how did it come to a decision.
Recently, Google made headlines when it medical AI failed to perform in the field. It was responsible for screening diabetes patients for diabetic retinopathy, which can cause blindness if not caught early. In the lab, it was great but in real life, the response was not so positive and nurses were not happy with the results – especially because they had no idea how a specific decision was made. This clearly shows us that our lab experiments need to leave the labs and be placed in front of humans for them to use it in the real-life for us to actually determine how useful they are. Even if a system fails, we should be in a position to pinpoint the cause of the failure and fix it as soon as possible. Today, when a system fails, the general answer is “I don’t know” and we want to change that.
Another example is inherent racial biases in today’s AI systems. Just because of how someone looks, we can’t attach stereotype especially when we’re deploying AI algorithms to help us decide. This is the area where we need to truly understand how the AI system came to this conclusion, whether the model is fair in treating sub-groups within the data, whether there was an issue collecting data in the first place. So pin-pointing to that specific area that needs improvement is highly critical and strengthens our case for explainable AI.

Additionally, the absence of explainability in black-box AI models creates hurdles for business growth and fails to add any real business value. It becomes more clear when we look at the business function as a whole: For the business decision-maker, we need to answer the question of why they can trust our models, for IT & Operation, we need to tell them how can they monitor and debug if an error occurs, for the data scientist, we need to tell them how they can further improve the accuracy of their models and finally, for regulators and auditors, we need to be able to answer whether our AI system is fair or not?
If we are able to achieve all these things, our decision-making ability will enhance exponentially and real business value will be achieved. We want to design our AI applications and systems to ensure they are:
- Explainable: we should have an explanation for decisions on multiple levels
- Compliant: we should be able to evaluate the model’s performance and measure it for compliance to help auditors and regulations trust our system
- Monitored: we should be able to monitor the performance and fairness of the models as they make decisions for us
- Debuggable: we should be able to diagnose and identify the root cause of failures, biases to remove errors thus improving model accuracy and fairness.
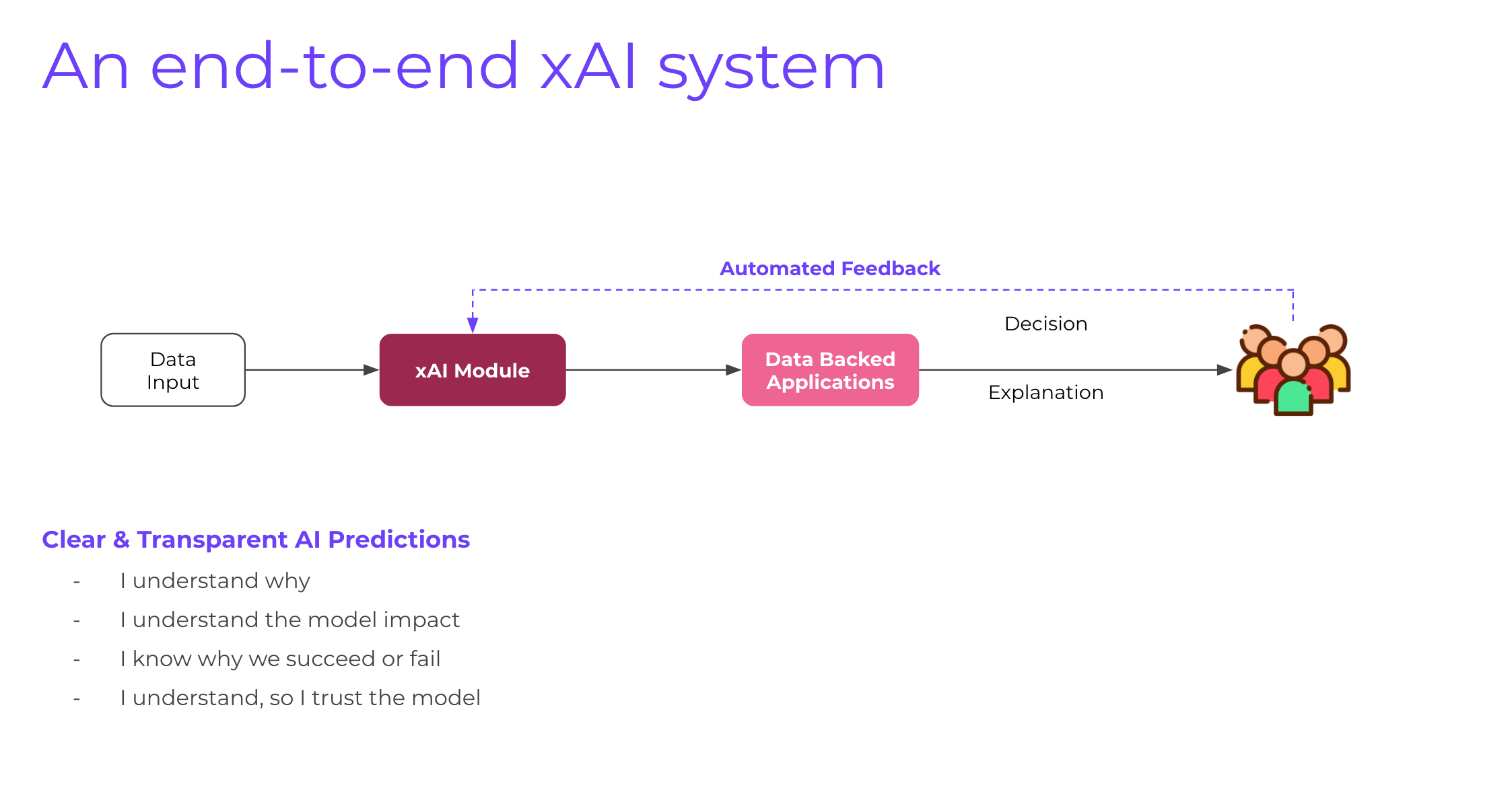
The need for XAI will only accelerate in the coming years
AI is positioned to transform every industry- from healthcare to financial services to law enforcement. The more involved AI is becoming, the more important it is becoming for us to understand and regulate its effect on us. The bad news is that even today, we have very little understanding of how AI systems arrive at these decisions. In summary, we don’t fully grasp the logic or reasoning behind AI decisions since many of the advanced algorithms are total black boxes. More than that, in industry, there is still a large trust gap and the inability of a data scientist to explain the results to a business stakeholder in a business-friendly language. As we move forward, we have to address these challenges. AI is becoming a crucial part of our lives and we must be able to trust the AI systems that are in place. For that trust to be possible, we must understand the decisions these systems make. We must also identify any inherent biases or errors that might create a downside for the business. With public interest growing in AI and regulations demanding a right to an explanation from industries that utilize AI systems, companies will have no choice but to update or adopt AI tools that will remove the black box in these algorithms, thereby improving explainability, mitigating bias, and improving outcomes for all.
Raheel Ahmad (https://www.linkedin.com/in/raheelahmad12/) is an AI researcher at NYU Tandon and is the cofounder of explainX.ai (https://www.explainx.ai/), an explainable AI startup that helps businesses build trustworthy, transparent, and unbiased AI systems.
Image Credits
Featured Image: Increasing reports on biases in AI systems (collage)
Links:
Featured Image: Black-box AI creates confusion & doubt
In-post Photo: Picture created by Krishnaram Kenthapadi, August 2019
Featured Image: End-to-End xAI system
In-post Photo: Picture created by Raheel Ahmad, June 2020